Deloitte AI Institute
How AI agents are reshaping the future of work
Expanded use cases
and impact from GenAI
View the report
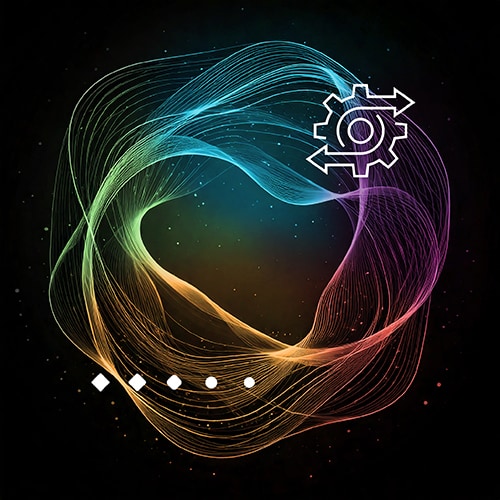
Key insights from this report
- AI agents are reshaping industries by expanding the potential applications of Generative AI (GenAI) and typical language models.
- Multiagent AI systems can significantly enhance the quality of outputs and complexity of work performed by single AI agents.
- Forward-thinking businesses and governments are already implementing AI agents and multiagent AI systems across a range of use cases.
- Executive leaders should make moves now to prepare for and embrace this next era of intelligent organizational transformation.
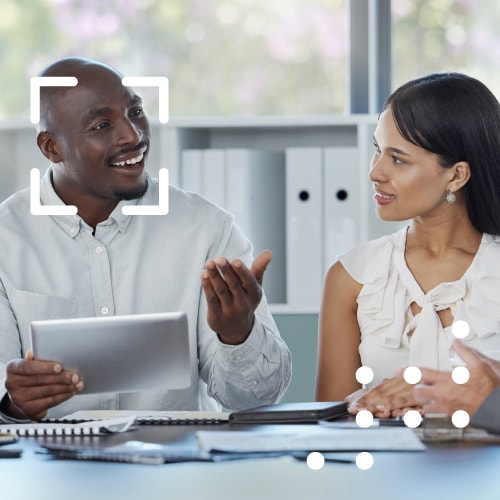
The next era of business process transformation is here
How can we operate faster and more efficiently?
This question has always been at the forefront of strategic agendas—but Generative AI (GenAI) is helping unlock new answers. Now the question for business and government leaders is becoming:
How can we rethink our business processes with GenAI?
Large language models (LLMs) and GenAI-powered tools used by most organizations today serve as helpful assistants. What if GenAI could be more like a skilled collaborator that will not only respond to requests but also plan the whole process to help solve a complex need?
This vision is becoming a reality with the emergence of AI agents and multiagent AI systems—a powerful advancement in what’s possible through human-AI partnership. Leading companies and government agencies are already seeing the value of AI agents and putting them into practice.
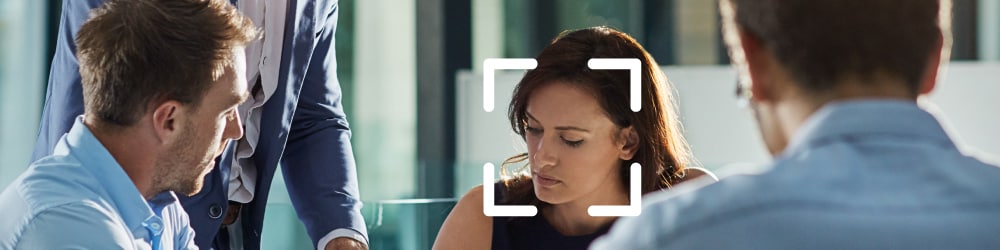
At the end of 2023, nearly 1 in 6 surveyed business leaders said GenAI had already transformed their businesses.1
What makes AI agents different—and why they matter
AI agents are reasoning engines that can understand context, plan workflows, connect to external tools and data, and execute actions to achieve a defined goal. While this may sound broadly like what standalone LLMs or GenAI applications can do, there are key distinctions that make AI agents significantly more powerful.
Typical LLM-powered chatbots, for example, usually have limited ability to understand multistep prompts—much less to plan and execute whole workflows from a single prompt. They also struggle to reason over sequences, such as compositional tasks that require consideration of temporal and textual contexts.
AI agents excel in addressing these limitations while also leveraging capabilities of domain- and task-specific digital tools to complete more complicated tasks effectively.
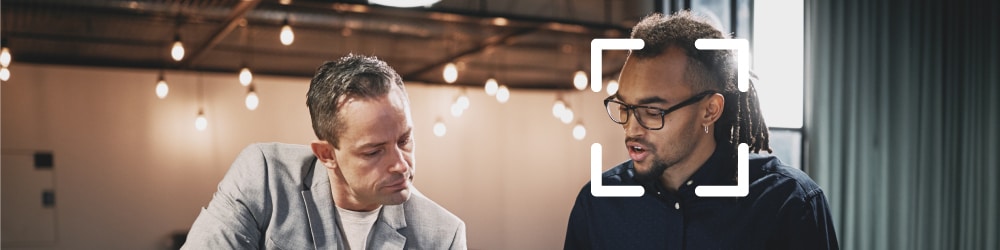
AI agents don’t just interact. They more effectively reason and act on behalf of the user.
A new paradigm for human-machine collaboration
Through their ability to reason, plan, remember and act, AI agents address key limitations of typical language models.
A new paradigm for human-machine collaboration
Through their ability to reason, plan, remember and act, AI agents address key limitations of typical language models.
Typical language models | AI agents | |
---|---|---|
Use case scope | Automate tasks | Automate entire workflows / processes |
Planning | Are not capable of planning or orchestrating workflows | Create and execute multistep plans to achieve a user's goal, adjusting actions based on real-time feedback |
Memory & fine-tuning | Do not retain memory and have limited fine-tuning capabilities | Utilize short-term and long-term memory to learn from previous user interactions and provide personalized responses; Memory may be shared across multiple agents in a system |
Tool integration | Are not inherently designed to integrate with external tools or systems | Augment inherent language model capabilities with APIs and tools (e.g., data extractors, image selectors, search APIs) to perform tasks |
Data integration | Rely on static knowledge with fixed training cutoff dates | Adjust dynamically to new information and real-time knowledge sources |
Accuracy | Typically lack self-assessment capabilities and are limited to probabilistic reasoning based on training data | Can leverage task-specific capabilities, knowledge and memory to validate and improve their own outputs and those of other agents in a system |
Multiagent AI systems: Amplifying the potential of AI agents
While individual AI agents can offer valuable enhancements, the truly transformative power of AI agents comes when they work together with other agents.
Multiagent AI systems employ multiple, role-specific AI agents to understand requests, plan workflows, coordinate role-specific agents, streamline actions, collaborate with humans and validate outputs.
Multiagent AI systems typically involve standard-task agents (e.g., user interface and data management agents) working with specialized-skill and -tool agents (e.g., data extractor or image interpreter agents) to achieve a goal specified by a user.
At the core of every AI agent is a language model that provides a semantic understanding of language and context—but depending on the use case, the same or different language models may be used by agents in a system. This approach can allow some agents to share knowledge while others validate outputs across the system—improving quality and consistency in the process. That potential is further enhanced by providing agents with shared short- and long-term memory resources that reduce the need for human prompting in the planning, validation and iteration stages of a given project or use case.
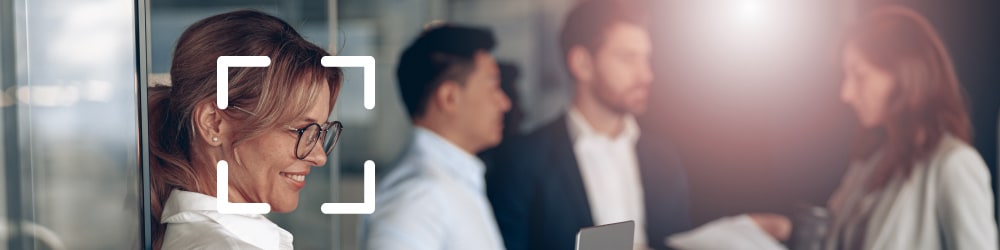
Multiagent AI systems don’t just reason and act on behalf of the user. They can orchestrate complex workflows in a matter of minutes.
Key benefits of AI agents and multiagent AI systems
- Capability—AI agents can automate interactions with multiple tools to perform tasks.
- Productivity—AI agents can plan and collaborate to execute complex workflows based on a single prompt.
- Self-learning—AI agents can rapidly improve their output quality over time.
- Adaptability—AI agents can reason and plan new approaches, rapidly reference new and real-time data, and engage with other agents to meet changing needs.
- Accuracy—“Validator” agents can interact with “creator” agents to test and improve quality and reliability.
- Intelligence—When agents specializing in specific tasks work together, new levels of machine-powered intelligence are made possible.
- Transparency—Multiagent AI systems enhance the ability to explain AI outputs by showcasing how agents communicate and reason together.
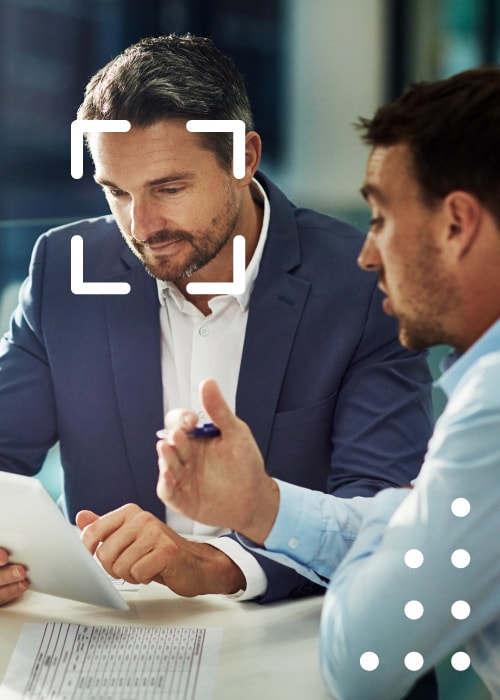
We see a future in which agents will transform foundational business models and entire industries, enabling new ways of working, operating and delivering value. That’s why it’s important for C-suite and public service leaders to begin preparing now for this next chapter in the evolution of human-machine collaboration and business innovation.
In our full report we explore four AI agent use cases that are possible today—two in specific industries (financial services and consumer) and two that can be applied in any organization. We discuss the future of AI agents and implications for enterprise strategy, risk, talent, business processes, technology and data. And we provide recommended actions to help leaders begin exploring initial applications / use cases of agents, while setting the stage for organizational transformation.
Effective and efficient work depends on creativity and knowledge augmented by well-planned processes and task-appropriate tools. That’s what AI agents and multiagent AI systems can bring together.
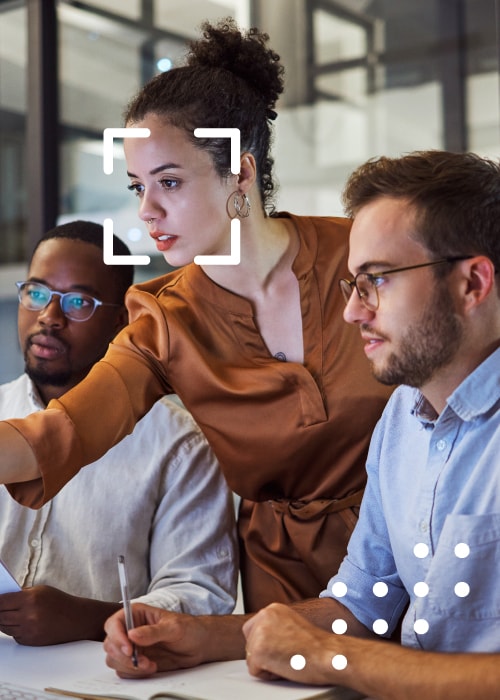
Prompting for action: A series on AI agents and multiagent AI systems
AI agents are opening new possibilities to drive enterprise productivity through business process automation. Use cases that were once thought too complicated for GenAI can now be enabled at scale—securely and efficiently.
In this series, we explore what makes AI agents so groundbreaking. And we share tangible recommendations to help C-suite leaders guide the journey for their own organizations.
Contributors to this report: Jim Rowan, Parth Patwari, Rajib Deb, Brijraj Limbad, Hye Ra Moon
Endnotes 1 Deborshi Dutt, Beena Ammanath, Costi Perricos and Brenna Sniderman, Now decides next: Insights from the leading edge of Generative AI adoption, Deloitte, January 2024, p. 8.