Finding the sweet spot has been saved
Article
Finding the sweet spot
Ultimate precision in store location is now a must for retailers
Where a store should be and what it should offer are increasingly complex decisions. Advanced analytics answering clear-cut business questions enables a precise location approach - using a smart combination of integrated data, clustering and predictive analytics techniques as the means to effectively translate information into action.
By Marit Keemink, Joris Vroegop and Leonora Lawson
Go directly to
- Determining business questions
- Answering business hypotheses
- Importance of clustering and benchmarking
- Case study
- Contact
Stores are under pressure. 2019 was a record-breaking year for the US with 6000 store closures . Dutch shopping streets have lost multiple prominent stores since the bankruptcy of V&D in 2015, with Intertoys, CoolCat, and Sissy Boy being the latest examples; meanwhile Amazon is preparing to enter the market. Yet, brick and mortar channels remain a first principle and compelling competitive advantage for many retailers. On average we see that when a new door opens, there is a 50% result in retailers’ sales growth in the surrounding area. Many consumers still want to directly see and touch the product, or be advised. Online retailers, such as Coolblue also use stores as the place to forge customer confidence in their purchase choice, while others such as Made.com and Lush create an experience that adds value to the product itself. Now more than ever, there is more pressure to get it right: where a store should be and what it should offer are increasingly complex decisions. Advanced analytics is the core enabler in a precise location approach - using a smart combination of integrated data, clustering and predictive analytics techniques as the means to effectively translate information into action.
Determining clear-cut business questions is the essential starting point
Most important is to first be clear about the location decision to be made and the information required to optimize for that decision. Without clear business questions to guide the analytics, the greatest risk is ending up with lots of data but no actionable insights. Be explicit about the level of insight required to make the choice actionable – if you want to analyze new countries or cities for expansion, you need data at this level, but if you want to optimize your store network within a city, you likely need data at the neighborhood or postcode level. If prioritizing markets for expansion, initial business questions could be: Where should I open my new store to be accessible to as many potential customers as possible? Or Where do I find the most potential customers who are attracted to my brand?. Hypothesizing one level deeper on what actually drives success is then key to pinpoint data indicators required: e.g. sales are higher in districts with universities and public facilities . With a business driver framework and information identified to make a proper trade-off (as well as hypotheses to test), data gathering can begin.
Smartly combine internal and external data that answer your business hypotheses
The most effective strategies integrate internal and external data sources across a legion of factors that drive performance. For example, external data on consumer behavior and demographics (population, age, disposable income, household size, internet access), as well as market dynamics (transaction volumes, traffic, points of interest such as supermarkets and metro stations, competitor locations, commercial developments) are highly relevant to gauge the potential. When combined with external data, internal data (sales, average spend, customer demand, customer origin, shopping behavior) becomes much more valuable, because it enables you to identify external drivers for performance. To enable analytics across sources, smart integration of the different data into one (cloud) platform is key.
Successful clustering and benchmarking leads to performance insight and precise predictions
To be able to quantify market potential and predict performance in areas without current presence, geographical clustering (segregating cities or neighborhoods into groups based on similar traits) is key. Next to that, it provides a means to compare performance of current stores and optimize accordingly. Clustering is data driven (based on the indicators identified by business hypotheses for distinguishing location success) and performed on external variables, such as those outlined previously describing the consumer profile and market. Clustered locations (segments) have very similar market dynamics and therefore allow comparison. Hence within a segment, a best performing location can be set as a benchmark for (potential) other locations. Also, it often turns out some segments perform better than others, which in turn allows you to discover distinct drivers (metrics distinguishing a segment) of performance. Estimates for market potential can be further refined by accounting for competitor density and cannibalization risk - identifying catchment areas and store saturation levels. It results in precise predictions for (future) market potential on an actionable level (city, neighborhood, or postal code area). While performing this modelling, it is crucial to have a dynamic approach and a setup that allows quick reproducibility. Because of this, you can easily scale from a local proof of concept to a more developed model for a greater coverage.

Identifying white spots based on a market potential heatmap, red indicates high market potential, round pointers denote current stores and yellow squares are points of interest.
Case study
To illustrate, one retailer implemented its four year country expansion plan across almost two thousand stores within less than a year due to prescriptive location strategies at neighborhood level. Such precision resulted from concrete business questions (Where should we have our stores and what format should they have?) and hypotheses to drive the data collection at postcode level (e.g. the more points of interest for tourism, the higher the sales). Clustering of geographies was performed across six external data sources containing a multitude of indicators (e.g. income levels, population density, retail locations, public transport availability, etc.). This clustering led to seven distinct neighborhood segments (e.g. premium destinations, residential zones, commercial centers, etc.). These segments were then used as the dominant lens to derive insights into market potential, channels, and products by performing in-depth analyses. Per geographical segment, current best performance was benchmarked to identify how many stores and which format (assortment, layout, etc.) would best capture the potential in every neighborhood. This also resulted in great insight into their existing network, suggesting which stores would actually benefit from format renewal, or indeed, closure. Combining all this, actionable city scenarios (which locations to open, close, or renew) were created. To foster business adoption, results were presented to country retail managers in the form of scorecards and playbooks to execute and monitor performance.
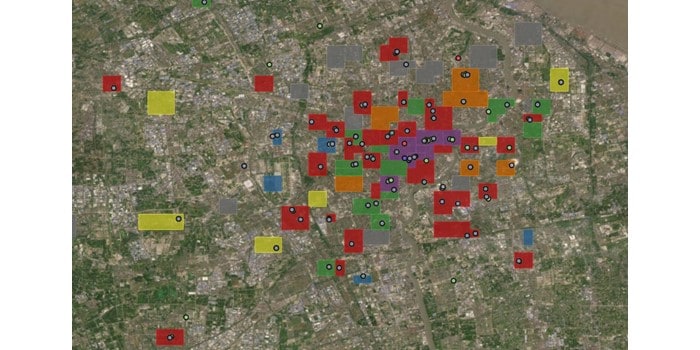
Neighborhood segment map: optimal store location and assortment
Although setting up a store for success starts with a holistic view on its purpose within the multi-channel network, with a business-hypothesis led and analytics-enabled approach we are able to shore up location strategy, making it accurate and actionable. (“Opening a new type A store in neighborhood X, with B assortment and pricing, will create an expected growth of Y% in revenue.”) The most effective approaches prioritize insight precision through identifying key predictors of success across a multitude of metrics, and further drive frontline decision making with granularity and intuitive presentation.
Organizations can generate value through analytics-driven strategic choices.
Read the Smart Growth blog seriesMore information?
For more information please contact Stefan van Duin, Perry van Overveld or Laurien Overakker via the contact details below.