Artikkeli
Generative AI deciphered
As technology evolves at an unprecedented pace, generative AI emerges as a transformative force, reshaping industries and influencing strategic decision-making. In this exclusive interview with Deloitte’s Analytics Leader Jouni Alin, we delve into the topic of generative AI, exploring why it holds paramount significance for the boards and other decision-makers in the corporate world.
In the recent Deloitte survey with European CFOs, Deloitte CFO Survey Autumn 2023, it was uncovered that only a fifth of companies in the EU see generative AI as either important or very important for their business and strategy. However, the survey also found that businesses are expecting there to be a potential benefit from an improved client experience and cost reductions due to generative AI. But in what way does it matter to boards of directors?
We asked Jouni Alin – partner in Consulting, and the leader of the AI & Data offering in Deloitte Finland – to answer a few questions on the relevance of this emerging and much debated topic. Our discussion appears below.
Thank you for speaking to us on this topic, Jouni. Now, there is much interest in AI and, in particular, in generative AI. Can we start with a basic question? How different are AI and generative AI?
Generative AI is a very specific type of AI. As the name ‘generative’ indicates, it does generate: it generates new content, a voice, text, code, video or image that is then at your disposal.
This is different from AI in general, which is already doing a wide range of everyday things, such as optical character recognition used in scanning texts or the license plate of your car, translating our speech into text or simply enabling Siri.
However, I say ‘new’ content, but generative AI needs something to feed itself on. Generative AI is typically based on a foundation model, transformer models learning about relationships in huge amounts of training data. There is a variety of these models that have usually been trained on a large amount of content from the public domain over some time. Some of these trained models have been made available as ‘open-source’ models, that is, models that you can freely pick up and use, given you have the infrastructure to run them in place.
Generative AI is not about creating something from nothing. AI is not an intellectual being – it is something that has been trained with data. Also, you need to feed it ideas and then it will generate something for you. It is a little like a sparring partner in that sense.
It is good to remember that what the generative AI foundation model has been trained on will have a big impact on what it is good for. For example, a colleague was helping his son with his homework using ChatGPT. After a while they realised that ChatGPT was pulling the information from a 'nonsense discussion forum' in Finland. In this example, there was one very large language model which was gathering also the content written in anywhere in public discussion forums over the internet.
You mentioned generative AI is ‘like a sparring partner’. How will our working habits change?
I can give you a very practical example of coding and conducting some data analysis: Microsoft has released something which is called GitHub Copilot, and others have similar offerings.
It’s trained on the public code repositories of GitHub – which equates to a globally-known, big developer community – where the public codes are available for anyone. Many organizations also publish their selected libraries of codes there that they are happy to offer to a wider audience.
GitHub Copilot also has a chat feature which allows a developer to ask questions such as ‘How do I transpose a matrix in Python?’ (which could be a question from someone who has not used much Python before). In turn, it will give you examples and codes.
Then, you have a common example of Q&A and a chatbot interface, which basically captures shown specific information provides a Q&A interface on top of it. It can help you find a firm’s holiday policies, company documents or even connect you to a co-worker, subject to shown information of course. These types of use will make our working life efficient because we are currently overwhelmed by the amount of available information.
Does the benefit of AI differ from company to company, and are there certain industries that benefit more than others?
In principle, all industries can potentially benefit, but there may be also other impacts for leadership to consider. Many companies are conducting analysis to understand what are the implications of GenAI for business as we see it today and also to understand the overall impact of AI in the long run, once it has become available for everyone.
We can certainly improve some aspects of business, but some activities may become redundant, particularly where AI is scaled up and taken into full use. This is likely to be work involving knowledge tasks, which is a large part of the work we do today.
Can you talk about impacts on internal or external stakeholders – particularly workers and their work in the future?
This is not a question that we can answer simply. If you think of knowledge work that is based on learning to do something and expanding that learning and repeating it or extending it – again, coding is one such example – while it would be wrong to say that generative AI will replace all the coders, it can make them much more powerful and productive than they are today. This can then have a big impact on the market. Data science and data engineering have been hot professions in the job market globally, but what will happen if productivity radically increases?
In other words, the monotonic part of our work will be radically reduced, which some people may be grateful for. If you can show how a repetitive piece of work is done in theory, you should be able to train an algorithm to do it. Then you can turn your focus onto building the algorithm and validating the output.
Training AI itself will, over time, become something that anybody can do. Maybe today it still requires somewhat technical capabilities and an understanding of what you are doing. But the whole idea of this foundation model is that it is trained with a vast amount of information which you can take and apply to your specific use, finetuning it further. This can be done by focusing on or providing the context from a certain subset. This is quite easy to achieve, and one could start training the model or calibrating the model towards it. This may sound a bit technical, but it will be more like ‘show me the training data, and I will give you the model’.
Talking about external stakeholders, let’s take an example of a financial advisor of a bank or a wealth management company. To act as someone in such a position, a person must possess knowledge of the regulations, financial products and vehicles, and go through extensive training. One can imagine that, at some point, it may become possible to train an AI customer service agent because AI is able to handle that type of information, even when it becomes too big or too complex for an individual human.
Today, the quality of chatbots as customer service agents requires us to consider our question formulation to optimize responses and obtain the desired information. But AI will improve and adapt to us.
Are there risks to AI?
Of course. We are already seeing some troubling use of popular social media platforms upon which people seek information or topics that can be harmful to them if not governed, monitored or regulated. Best practice and transparency are also needed in order to provide us with healthy outcomes from the use of AI.
What is the role of the board? Are there skillsets they will have to fulfil their role? And, if so, how can they do this? When does it become necessary to bring in a specialist to the board, given that a board cannot accommodate very many experts?
Regulations may follow, but concerning AI, it is hard for companies and organisations to be reactive. There’s no ‘put this on hold’ type of option.
Whether you like it or not, your employees, your people, are going to try it out – and that may be the least of your concerns. They might also do some business with it, apply it for some business-use cases.
Companies will need to start educating their employees and sharing their knowledge about risks, as well as exploring possibilities and developing ways with which to pick up the most prominent use cases and empower employees. Organisations need to drive the use of AI further and not close their eyes to what is happening (as this is simply unrealistic).
Many organisations are experimenting and are eager to develop packages and policies on AI use. They are looking to find ways to educate their employees and ways to provide support.
In this sense, the board’s considerations in regard to how to approach AI may not be any different from the way in which they approach any other new technology. They should consider what is happening now in the marketplace and our behaviour. They should manage change and prepare for the impact on our business and our ways of working.
To address these matters, do we need start upskilling our employees, shaping them into different roles? Do boards also need to acquire new skills? Perhaps. Human aspects, talent management and change management are big agenda items that boards need to consider in addition to technical upskilling.
Do boards need specialist input?
Specialists may help in igniting the thinking process and getting the discussion started in the boardroom. Experts may also be well suited to assessing the impacts on the organisation from the market perspective. But the responsibility should not fall on the shoulders of one person – in that sense, it is similar to digital transformation which will impact all.
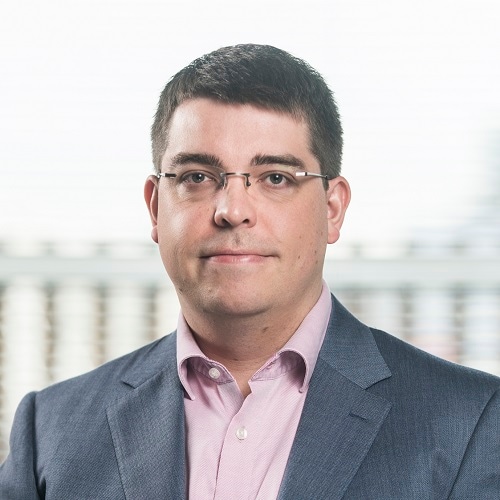