Forensic analytics in fraud investigations has been saved
Perspectives
Forensic analytics in fraud investigations
Identifying rare events that can bring the business down
Forensic analytics combines advanced analytics with forensic accounting and investigative techniques to identify potential rare events of consequence—needles in the massive haystacks of data and information that can signal trouble in the making. Urgently needed to meet growing regulatory and customer demands for fraud mitigation, forensic analytics can reveal signals of emerging risks months or even years earlier than possible otherwise.
Explore content
Forensic analytics resources
Detection of fraud schemes has long involved searching for patterns in behavior, actions, relationships, and the movement of money. Forensic analytics helps organizations identify, thwart, and prevent attacks by integrating artificial intelligence (AI)-based data analysis with skilled forensic investigation of fraudsters' motives and methods.
In addition to its fraud-fighting applications, forensic analytics can be used to address operational issues, such as how an organization's processes and controls can create vulnerabilities as well as how they respond to evidence of possible issues. For example, one automaker discovered that, on average, defects could have been identified a year and a half earlier with a forensic analytics approach.
Methods employed to address these challenges include:
- An analytics repository integrates disparate data sources so analytical models can identify and consolidate signals from across an enterprise.
- Supervised modeling involves development of algorithms that articulate similarities between groups of historical fraud patterns, and identifies what separates them from the rest of the data population. For example, regression equations, decision trees, and neural networks can be designed to classify historical instances of fraudulent actions as being either high or low risk.
- Text and computer vision analytics are increasingly valuable investigative tools amid the explosive growth in unstructured data, including emails, messaging, audio, and video.
Analytic deployment considerations
Several methods warrant consideration in developing and applying forensic analytics:
- Training and self-learning. Analytics can learn from a variety of data sources, such as risk issues the organization has confronted in the past.
- Backtesting. Organizations can scientifically test forensic analytics performance in determining whether to use it.
- Iterative approach. As a forensic analytics solution is being implemented, models can be iteratively developed, adapted, and scaled so they respond to new and evolving fraud patterns, and at the same time, continually gain a broader view of the risks an enterprise may face.
- Feedback and continuous improvement. Once the forensic analytics solution is in place, its effectiveness can be continually improved by incorporating feedback from results of each investigation, from the continually growing body of forensic accounting and investigation knowledge and insight, and from the input of stakeholders across the enterprise.
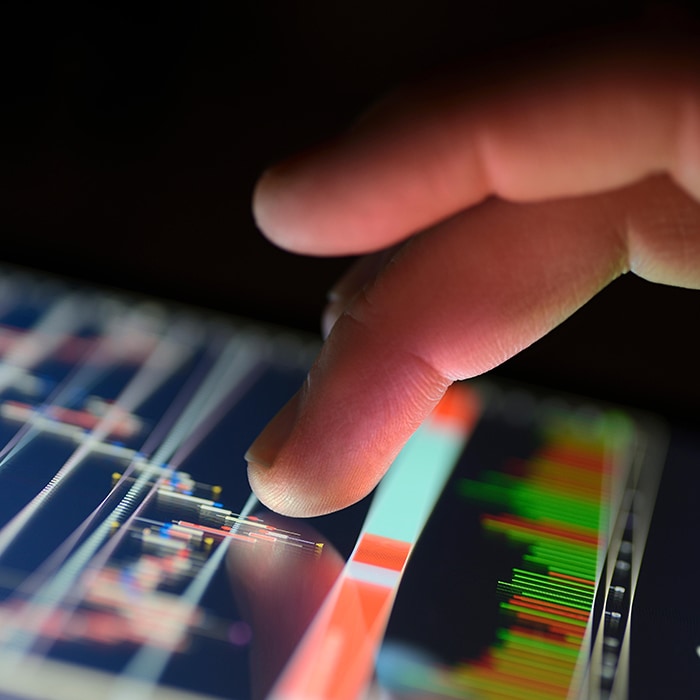
Recommendations
Five insights into anti-corruption compliance programs
Revisiting the FCPA
Cognitive computing applications for risk management
Better decision making with artificial intelligence