Deloitte AI Institute
AI in Post-Sales Customer Experience
Driving Superior Outcomes with AI for Customer Success
View the report
Current challenges in post-sales customer experience
To fully realize benefits from AI technologies, companies must begin by understanding the key challenges and productivity blockers that exist today. Between 2022 and 2023, we surveyed over 200 Customer Support and Success professionals and interviewed more than 25 senior leaders across 15 large enterprise companies. This research enabled us to uncover themes across both areas of Customer Support and Success that impair productivity and drive inefficiencies within the teams today.
At a higher level, significant opportunity exists for companies to enhance the post-sales customer experience and increase loyalty, satisfaction and trust with their customers. While 90% of end customers interact in some way or form with Customer Success teams, only 1/4th of them indicated that they consider their service providers to be trustworthy and would advocate for them unequivocally. On the Customer Support front, ~60% of end customers were not ‘highly’ satisfied with their support experience.

Customer support
Customer success
Leveraging AI to address post-sales experience challenges
The collective analysis of our study revealed one overarching insight—driving more automated and proactive motions across both Customer Support and Success using AI capabilities can enhance efficiencies and free up capacity for teams to focus on true customer value additive activities.
The advancements in AI technology, particularly Generative AI, over the last one year can significantly add value for these teams by automating many tasks performed manually today and eliminating productivity blockers.
Complemented with efforts to enhance data quality and increasing application of predictive AI and analytical models, companies can significantly empower their Customer Support & Success teams with more customer knowledge at rapid speed, reduce burden of effort and allow customer facing Customer Relationship Management (CRM) and Support reps to engage, define and enhance the experience.
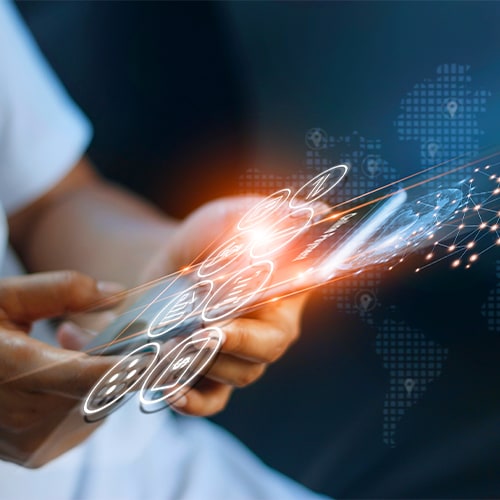
Core tenets
As companies look to embark on this journey, we believe they can anchor their efforts on five core tenets. By evaluating opportunities against these tenets, companies will be able to better understand how AI can enhance value and ultimately drive greater customer satisfaction, adoption, and retention. <br><br><strong>The five tenets that an AI enabled interventions for post-sales experience should fulfill include:</strong>
Core tenets
As companies look to embark on this journey, we believe they can anchor their efforts on five core tenets. By evaluating opportunities against these tenets, companies will be able to better understand how AI can enhance value and ultimately drive greater customer satisfaction, adoption, and retention.
The five tenets that an AI enabled interventions for post-sales experience should fulfill include:
Personalized and customer centric:
Drive a higher degree of personalization in the customer’s experience
Adoption and value oriented:
Enable customers to maximize adoption, usage and value realization
Improving worker productivity:
Automate routine processes, drive higher productivity and enable cost-effective omni-channel engagement
Augmented human element:
Surface insightful recommendations to enhance human engagement and interaction
Proactive and predictive:
Anticipate customer needs based on existing usage patterns and suggest the 'next best action'
Designing use cases to address challenges and realizing benefits
Based on findings from our research and our deep expertise in enterprise business performance and AI technologies, we have identified a set of use cases that companies can consider to address each of the challenges the survey presents. These AI driven use cases within Customer Success and Support functions can enable enterprises to address critical business challenges, achieve operational efficiency, optimize decision making and drive sustained growth.
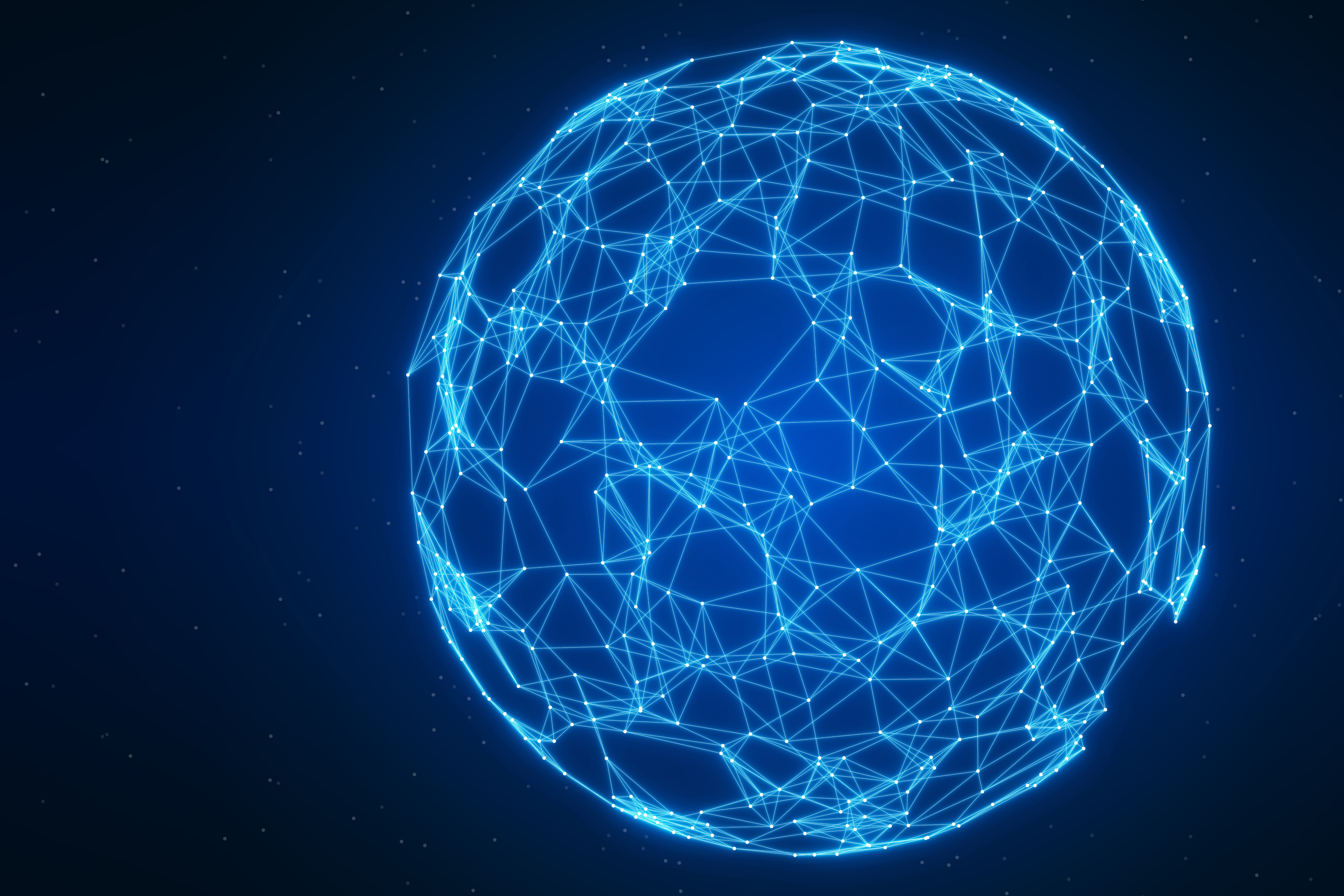
Customer support use cases
For Customer Support, the use cases primarily focus on improving resolution time, reducing cost-to-serve, enhancing self-serve capabilities and improving internal productivity of support teams.
Customer success use cases
For Customer Success, the use cases primarily focus on reducing manual effort through generative AI technologies and production of predictive insights to drive more proactive actions by CSMs on customer accounts to enhance experience and value realization.
Building a customer outcomes acceleration architecture
Within the space of Customer Success, AI capabilities can also help drive proactive journey orchestration for customers themselves. Companies can combine data from multiple systems such as CRM tools, financial systems, product consumption data, product telemetry data and customer survey/feedback data and add a layer of analytical and predictive AI capabilities on top of it. This can then take the form of a Customer Outcomes Acceleration System that can have customer facing console allowing them to:
- View how far they are along their product journey
- Get insights into their usage and how it compares to benchmarks
- Manage ownership of all their assets in one place
- Get actionable steps for increasing value realization
- Obtain personalized recommendations for usage and training
Such a capability can showcase all the relevant information for a customer in an integrated manner and place adoption and value realization in the hands of a customer themselves.
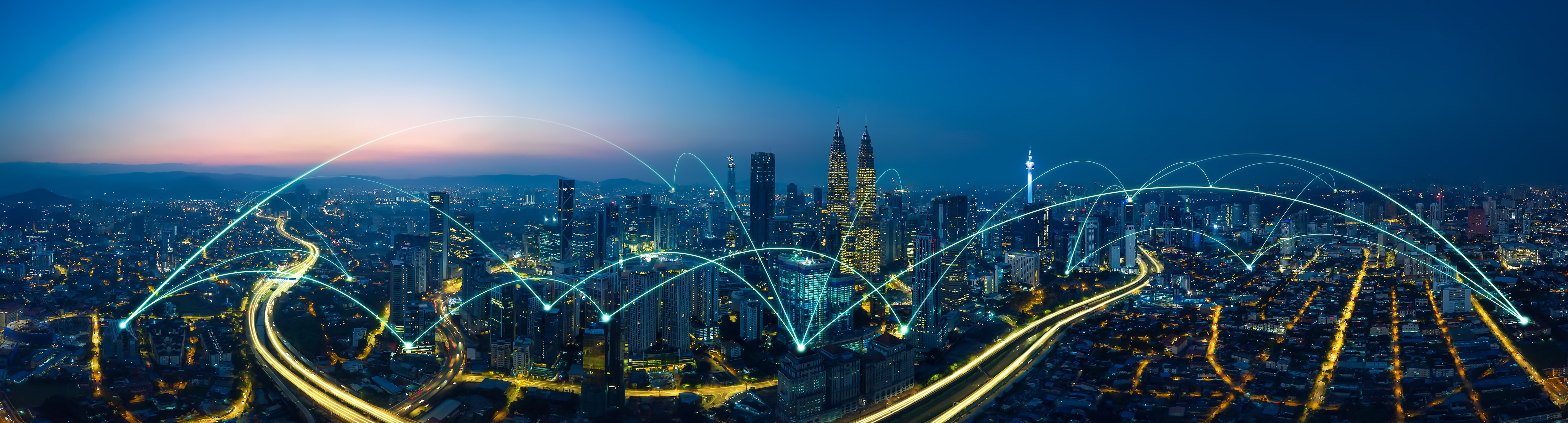
Getting started: Use case prioritization
As enterprise companies consider the above set of use cases, they can evaluate and prioritize them based on core objectives served and complexity of AI technologies involved. We believe that AI use cases will progress and can be implemented along a spectrum of three levels:
- Productivity enhancements - Enhance productivity with relatively simple use cases across Customer Support and Success using generative AI technologies (e.g., creating summary account views, writing knowledge articles, summarizing meeting notes and support ticket information).
- Predictive insights - Layer predictive insights on top of content produced by Generative AI tools. For example, predictive analysis based upon customer health and usage patterns and support ticket escalations can help derive insights from existing customer information in order to sequence actions.
- Proactive actions - Combine generative and predictive AI techniques to prompt the next best set of proactive actions. These use cases will hinge on the premise of anticipating issues before a customer becomes aware of them and proactively resolve them before they are surfaced or escalated. The AI helps support teams analyze telemetry and network data to flag any known inter-operability issues. AI can prompt Customer Success teams with a specific playbook to drive higher usage of the product based on usage patterns or it can help a network team proactively handle a hardware issue with maintenance to avoid an outage.
Getting started: Key success factors
To realize the above promise and deliver a superior post-sales customer experience through AI, enterprise companies will need to stand up a cross-functional, centrally coordinated effort that address five key dimensions. These include:
Getting started: Key success factors
To realize the above promise and deliver a superior post-sales customer experience through AI, enterprise companies will need to stand up a cross-functional, centrally coordinated effort that address five key dimensions. These include:
Technology:
Driving organizational alignment on key questions including:
- Post-sales technical architecture to support AI
- Build vs. buy assessment with regard to generative AI solutions; how much can be sourced ‘out of the box’ from vendors compared to where in-house capabilities need to be built for specific use cases
Talent:
Mapping the impact of AI on existing workforce with respect to:
- Upskilling required in the near to medium term
- Management of change and messaging with regard to rollout of AI
- Focusing current workforce on highest impact use cases in near term to mitigate risk of scarcity of AI skilled talent
Operations:
Establishing an AI Center of Excellence (CoE) that can:
- Streamline multiple AI efforts across teams to avoid AI sprawl and high costs
- Define common standards of governance, build and acceptance
- Defining clear prioritization criteria to identify highest value use cases
- Oversee pilot, test and launch efforts and secure commitment and participation from various team as needed
- Managing AI investment decisions in relation to the desired business outcomes and challenges
Data and platforms:
Ensuring data readiness for activating use cases through:
- Identifying key data elements required for individual use cases
- Streamlining and labelling data elements in data warehouse
- Channeling data elements from source data hubs into structured and unstructured data sets
Risk and compliance
Addressing regulatory, security and ethical concerns through:
- Defining a set of AI policies that address organizational and functional needs (e.g., tool access, prompt controls, keyword filtering etc.)
- Outlining parameters for data privacy, transparency for usage and IP protection
- Building methods for ensuring compliance with regulatory guidelines and standards
In conclusion, the future of customer experience is poised to be revolutionized by the vast potential of AI. By harnessing the power of artificial intelligence, businesses can unlock superior customer outcomes in their customer success and support efforts. Embracing AI now will position businesses at the forefront of the AI revolution, delivering unparalleled customer experiences and achieving long-term growth and competitiveness in a dynamic customer-centric landscape.
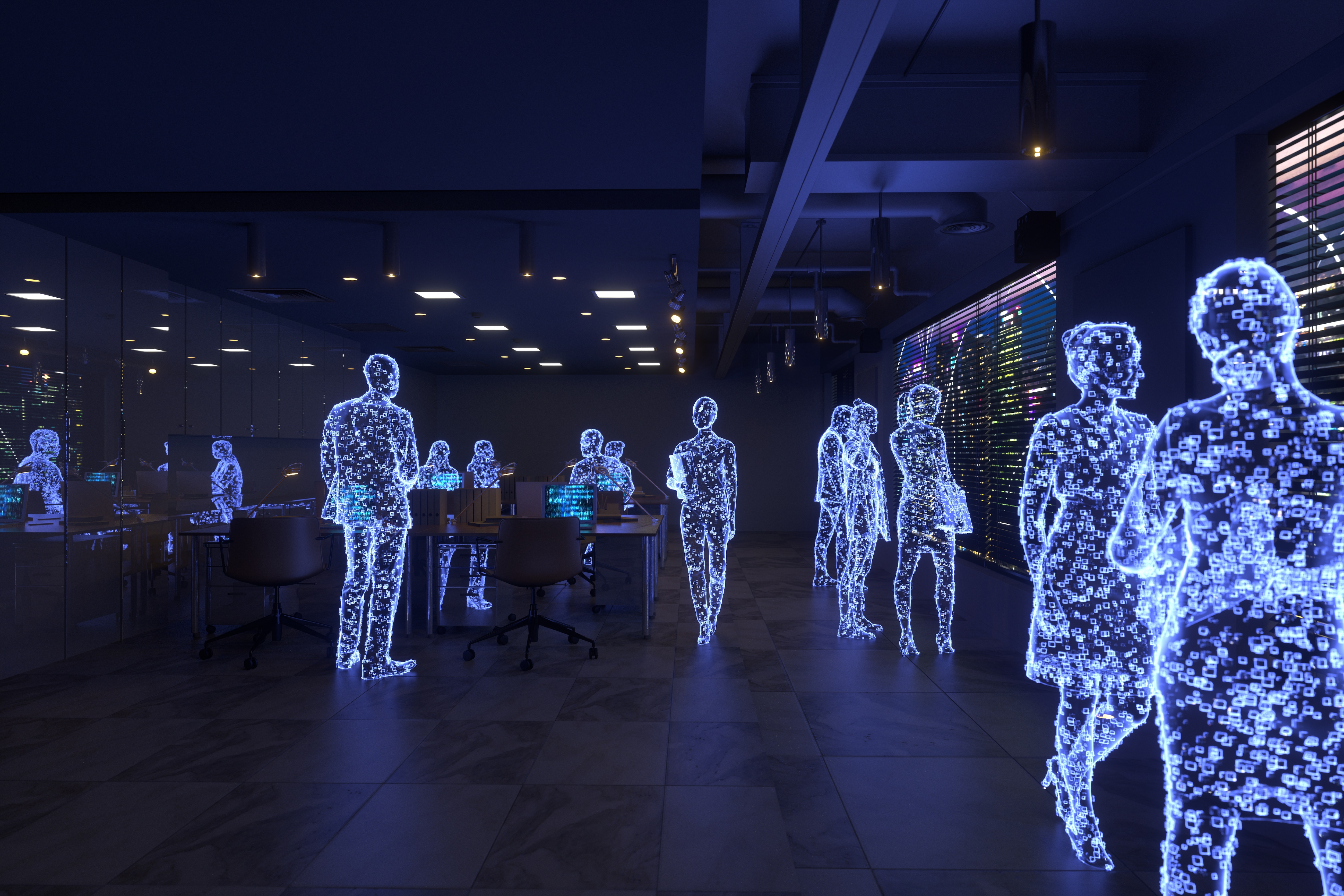