Fair Lending has been saved
Services
Fair Lending
Bringing first person know-how to predictive analytics
Predictive analytics is the practice of extracting information from existing data sets to determine patterns and trends. The data cannot tell the future, but it can give you a good idea of what to watch out for. When a lender understands that current practices translate to impacts it will be liable for tomorrow, the practice of taking a methodical look ahead can help protect it and its customers from unintended consequences. The very act of conducting predictive analytics can speak to a lender’s good faith and desire to comply with consumer compliance regulatory requirements.
Explore content
- Giving banks a fair chance to manage fair lending requirements
- What predictive analytics has to offer
- Common considerations
- Fair and responsible banking
- How we can help
Giving banks a fair chance to manage fair lending requirements
In central New York1, a mere complaint about fair lending practices—not even a regulatory action—is enough to put a bank merger on hold and put jobs on the line. In Washington, DC, the Department of Justice and the Consumer Financial Protection Bureau (CFPB) have joined forces to impose a $9 million consent order on a wholesale lender—based not on its actions, but on the actions of third parties. In the justice system, the Supreme Court has just affirmed disparate impact as a basis for housing discrimination claims, regardless of explicit intent. How can lenders not only make good on their intent to operate fairly, but also demonstrate their compliance in a way that wards off fines and reputational damage?
The root objective in fair lending is to be fair – to avoid doing the wrong thing, intentionally or otherwise. For a lending institution that values its customer relationships, it is good business to establish beyond a doubt that it operates with integrity. The current regulatory regime and environment that surrounds fair lending makes the stakes even higher for lending institutions. The tangible cost of fair lending noncompliance can be measured through fines and their associated legal costs. Even without the motivation of mitigating complaints and/or violations, it is extremely unwieldy and costly to run a compliance program that is operating blindly due to its incorrect use of data. Add the long-term cost of reputational damage and the risk of stunted growth due to poor Community Reinvestment Act (CRA) ratings, it becomes clear that fair lending is an area that merits a significant deal of care.
However, the news is not all negative. Consider that it costs lenders time and money to aggregate data for the purpose of satisfying compliance requirements. Right now, many of them send that value out the door not realizing that this same data can yield long-term value if lenders learn to mine it for insights into their customers and apply those insights to more consumer-centric business practices.
1 http://www.syracuse.com/business-news/index.ssf/2015/06/community_bank_oneida_savings_delay.html
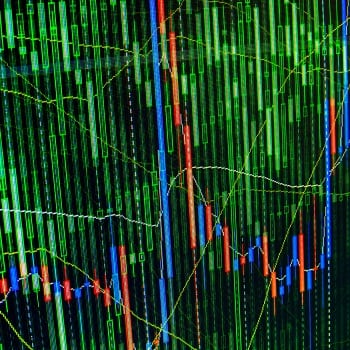
What predictive analytics has to offer
The best policing is often policing that comes from within. So many of the discriminatory practices we observe are preventable if the lender had proper internal controls. That work is vital to ensuring fair and2 equal treatment for borrowers.3
— Thomas Perez, Assistant Attorney General, US Department of Justice
From a fair lending perspective, institutions can run analytics on existing loan portfolios to understand the level of fair lending risk exposure. Running a statistical analysis may uncover areas of potential concern, which allows management to conduct further analysis before regulators are able to rule on a fair lending issue. Monitoring and self-remediating in this fashion can keep an institution a step ahead of unforeseen issues or future violations: Importantly, the practice can decrease the potential for disparate treatment against a protected class. Additionally, the lender that moves to police itself may appear more favorable in the eyes of regulators and regulatory agencies. The preemptive act of self-remediation will aid in avoiding a Matter Requiring Attention (MRA), a consent order, or a settlement; which will protect value and brand.
2 http://www.aba.com/aba/toolbox/fairlending/fairlending_tool1r.pdf
3 Assistant Attorney General Thomas Perez. Justice Department Annual Report and Congressional Testimony, December 2011
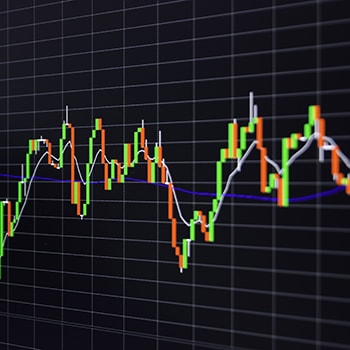
Common considerations when performing an analysis
Deciding to employ predictive analytics is only the beginning of a process. As with any complex tool, analytics require preparation and understanding to deliver on their full potential. When delving into statistical analysis, the preferred method for large datasets is the use of a regression model.
Running this analysis can be divided into seven core dimensions:
1. Establishing the scope
Management should begin by understanding what population it wants to analyze— and for what attribute. This decision may be driven by emerging regulatory concerns, the desire to perform an internal spot check on a portfolio, or as part of an ongoing monitoring program.
2. Selecting the dataset
A meaningful analysis starts with a large, complete, and robust dataset. In many institutions, multiple legacy servicing systems or systems of record can make it a challenge to attain a full population for a portfolio or type of product. The datasets should be governed by a strong control environment that tests for data accuracy and data integrity on a regular basis to evaluate if the data used in the model is accurate. Statisticians have an old saying: “garbage in, garbage out.” The model and its results are only as good as the quality of data used to run them.
3. Developing the model
There are myriad statistical methods and systems that lenders can use to develop models. It takes a trained statistician to understand the data, understand the objective of the fair lending analysis, and develop the model accordingly. A well-conceived model can control for certain borrower attributes like a FICO score, loan to value, and debt to income. The question it attempts to answer is: “With all else held equal, are borrowers from a protected class receiving different terms from the non-protected class?”
4. Geocoding the data
Home mortgages should be geocoded as part of the Home Mortgage Disclosure Act (HMDA) requirements. For other lending products, institutions may also choose to geocode data to run a meaningful fair lending analysis. Small populations can be geocoded manually by using the Federal Financial Institutions Examination Council’s (FFIEC) website. For large datasets this process can be very laborious and time consuming; nonetheless it can be outsourced to a third-party vendor who can efficiently and automatically geocode the population.
5. Establishing borrower government monitoring information (GMI) proxies
To run a fair lending analysis, all loans should have protected and nonprotected class information associated with them in a GMI format. For loans that are HMDA reportable, this information should already be provided. For non-HMDA reportable loans, proxy information will have to be used. The CFPB released a methodology in 2014 that favors the Bayesian Improved Surname Geocoding (BISG) proxy method,4 which combines geography- and surname-based information into a single proxy probability for race and ethnicity.
6. Reviewing results and matched pair analysis
After the model is run, certain areas (such as specific products, processes, or services) are likely to require further analysis. After this subset has been identified, matched pair analysis between borrowers with similar characteristics may be conducted. In matched pair analysis, lenders identify similarly situated borrowers of a protected and non-protected class who have applied for similar loan products but with differing outcomes. The comparison is made to determine if evidence of potential disparate treatment is present. Matched pairs should be selected in accordance with the Interagency Fair Lending Examination procedures Appendix V: “Identifying Marginal Transactions.”5
7. Understanding the data
This may seem intuitive, but it can be deceptively misleading. Understanding what the data consists of, its statistical distributions, and its unique characteristics before, during, and after developing a model will help establish a level of comfort around the approach as well as the results.
4 Consumer Financial Protection Bureau. (2014). Using publicly available information to proxy for unidentified race and ethnicity. Retrieved from
http://files.consumerfinance.gov/f/201409_cfpb_report_proxy-methodology.pdf
5 http://www.ffiec.gov/PDF/fairlend.pdf
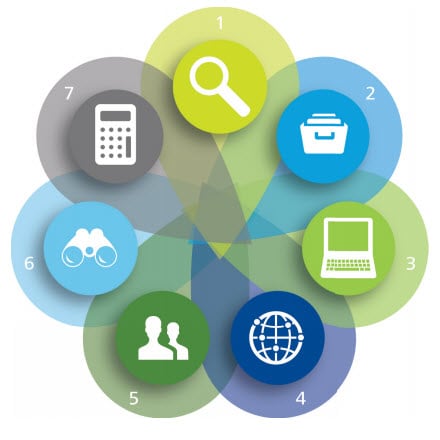
Considerations table of contents:
5. Establishing borrower government monitoring information proxies
6. Reviewing results and matched pair analysis
Fair and responsible banking: A requirement, but also a goal
As the transition from fair lending to fair and responsible banking takes place, statistical analysis presents additional opportunities that go beyond compliance. Responsible banking practices can keep a lender out of trouble and increase efficiencies in an institution’s operations, improve customer relations, and fine-tune the customer experience.
For example, a lender can apply statistical analysis to consumer complaints. Complaints could be broken out by complaint type or regulatory applicability and then analyzed further to understand fair or responsible banking implications. Perhaps there is a higher instance of a certain complaint type primarily originating from a metropolitan statistical area (MSA) with a high concentration of a protected class. This information could help shape outreach programs to those borrowers, reduce fair and responsible banking risk, and potentially support the institution’s Community Reinvestment Act (CRA) strategy.
A bank that engages in these targeted activities can use data it already paid to amass—resources that were previously earmarked only for compliance—to build its brand and improve customer experience.
How Deloitte Risk and Financial Advisory can help
Predictive analytics is a specialized discipline that many offer but at which few excel. Banking and lending are complex activities that make up only one part of a multifaceted financial services industry. The practice of regulatory compliance is an art and science all its own. There is no shortage of third-party allies ready to help; however, there are very few that can bring experience and a record of accomplishment to the table. Deloitte Risk and Financial Advisory’s Fair Lending practice group includes industry veterans and former regulators who bring first-person know-how to this complex challenge.
Contact us
For more information about Deloitte's Fair Lending services, please contact any of the following Deloitte professionals:
Tamara Milliken
Managing Director
Deloitte Risk and Financial Advisory
(704) 887 1876
Maria Marquez
Senior Manager
Deloitte Risk and Financial Advisory
(415) 378 0751
Konstantine Loukos
Senior Consultant
Deloitte Risk and Financial Advisory
(703) 251 3795
Recommendations
Financial Services RegPulse Newsletter
Concise summaries of select regulatory developments
Rethinking compliance management
For banks