Deloitte AI Institute
Building Trustworthy Generative AI
Responsible generative AI ethics and security is the core of safety in this new AI frontier
View the report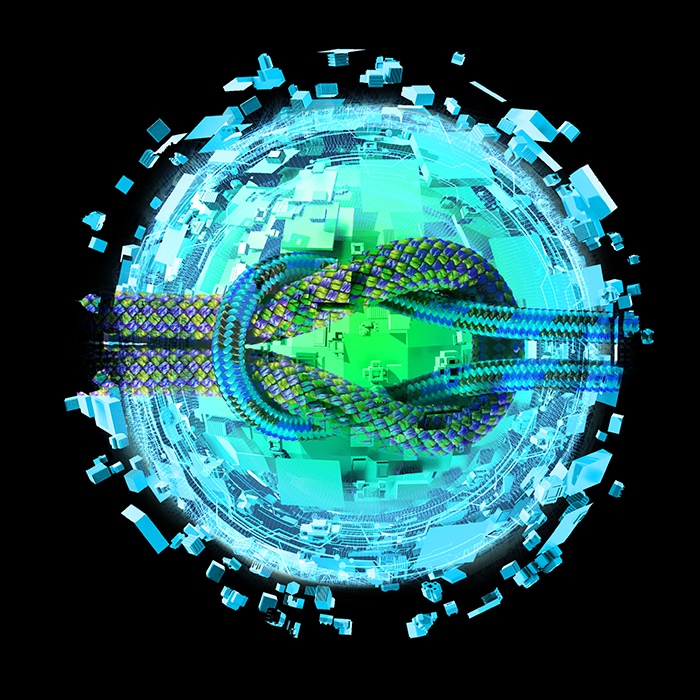
This Fragment is the AI Institute Sidebar
This text and the space it occupies will not be visible in the following environments: "View as published", "Preview", "Live"
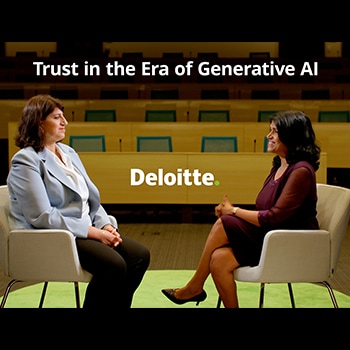
Large language models and other types of generative AI have thrown open the door to capabilities many assumed were still in our future.
Yet, as organizations explore this new technology, the glimmer is giving way to the practicalities of using generative AI for business benefit, and it is in generative AI risk mitigation and governance that we see a need for action.
For all the attention and investment in generative AI research and development, there has not been commensurate investment in addressing and managing the risks.
Fortunately, we are not starting from scratch. While generative AI is new in many ways, it accelerates and amplifies risks that have always been a factor in AI development and deployment. The concepts and tools that enable Trustworthy AI still apply, even as many of the emerging risks and problematic scenarios are more nuanced.
To prepare the enterprise for a bold and successful future with generative AI, we need to better understand the nature and scale of the risks, as well as the governance tactics that can help mitigate them.
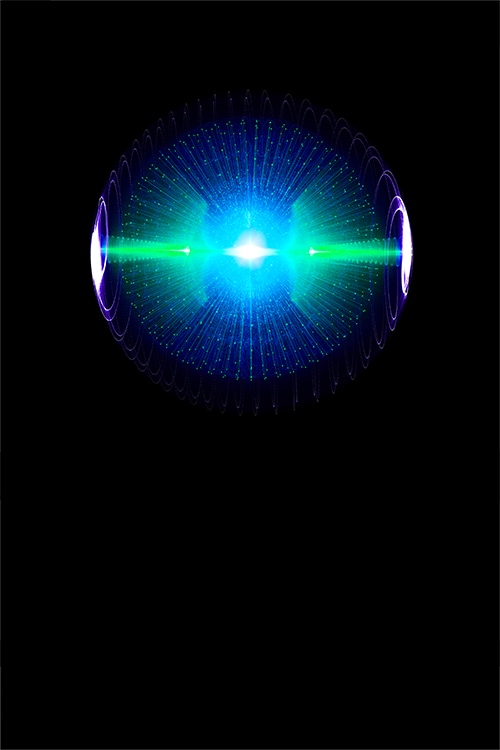
Mapping trust domains to Generative AI
In discussions of generative AI, one type of risk is commonly noted: hallucination. Generative AI models are designed to create data that looks like real data, but that doesn’t mean outputs are always true. Sometimes, the model takes a wrong turn. While generative AI hallucinations can be a hurdle significantly impacting user trust, they can be mitigated by considering it as a model reliability issue. But then, it is not the only (nor even the most significant) risk.
Taking a broader view, we can use the trust domains in Deloitte’s Trustworthy AITM framework to explore the types of risks with which organizations may contend when deploying generative AI. Trustworthy AI (of any variety) is: fair and impartial; robust and reliable; transparent and explainable; safe and secure; accountable and responsible; and respectful of privacy.
While not every trust domain is relevant to every model and deployment, the framework helps illuminate generative AI risks that deserve greater concern and treatment.
${pullquote}
Copyright © 2022 Deloitte Development LLC
Fairness and impartiality
Limiting bias in AI outputs is a priority for all models, whether machine learning or generative. The root in all cases is latent bias in the training and testing of data. Organizations using proprietary and third-party data are challenged to identify, remedy, and remove this bias so that AI models do not perpetuate it. This is not just a matter of unequal outcomes from AI-derived decisions.
For example, a generative AI-enabled chatbot that produces coherent, culturally specific language for an audience in one region may not provide the same level of nuance for another, leading to an application that simply performs better for one group. In practice, this could diminish end user trust in the tool, with implications for trust in the business itself.
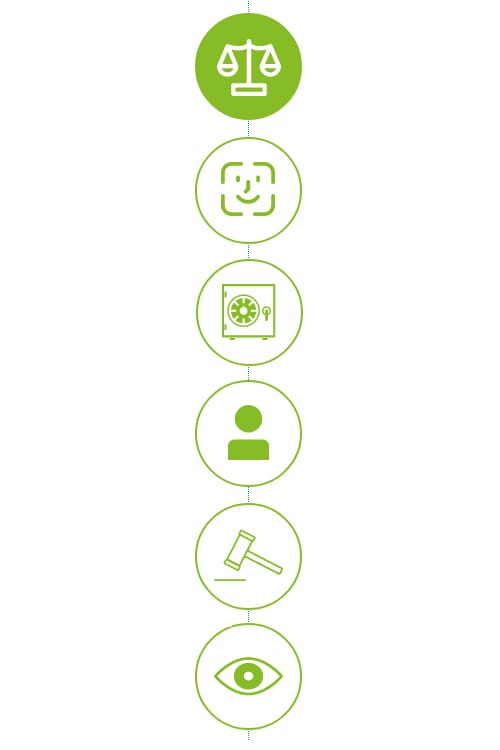
Transparent and explainable
Given the capacity for some generative AI models to convincingly masquerade as a human, there may be a need to explicitly inform the end user that they are conversing with a machine. When it comes to generative AI-derived material or data, transparency and explainability also hinge on whether the output or decisions are marked as having been created by AI.
For example, a generative AI-created image may require a watermark to indicate its AI origin. Similarly, in the healthcare arena, a medical recommendation made by a generative AI system may require notation that it was machine derived, as well as accessible, digestible logs or explanations as to why that recommendation was made.
More broadly, to trust the model and its outputs, stakeholders within the enterprise, as well as end users, need an understanding of how input data is used, an opportunity to opt-out, obscure, or restrict that data, and an accessible explanation of automated decisions and how they impact the user.
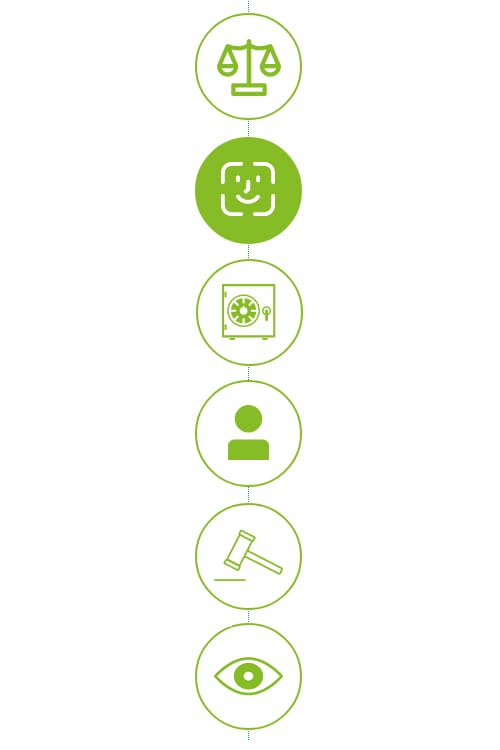
Safe and secure
Powerful technologies are often targets for malicious behavior, and generative AI can be susceptible to harmful manipulation. One threat is known as prompt spoofing, wherein an end user crafts their inputs to trick the model into divulging information it should not, not unlike how traditional AI models are targeted for reverse-engineering attacks to reveal the underlying data. In addition—particularly given generative AI’s capacity to mimic human speech, likeness, and writing—there is a risk of massive misinformation creation and distribution.
Generative AI can permit near-real-time content personalization and translation at scale. While this is beneficial for targeted customer engagement and report preparation, it also presents the potential for inaccurate, misleading, or even harmful generative AI-created content to be disseminated at a scale and speed that exceeds the human capacity to stop it. A generative AI-enabled system could erroneously create products or offerings that do not exist and promote those to a customer base, leading to brand confusion and potentially brand damage.
More troublingly, in the hands of a bad actor, generative AI content could be used maliciously to create false or misleading content to harm the business, its customers, or even parts of society. To promote generative AI safety and security, businesses need to weigh and address a myriad of factors around cybersecurity and the careful alignment of generative AI outputs with business and user interests.
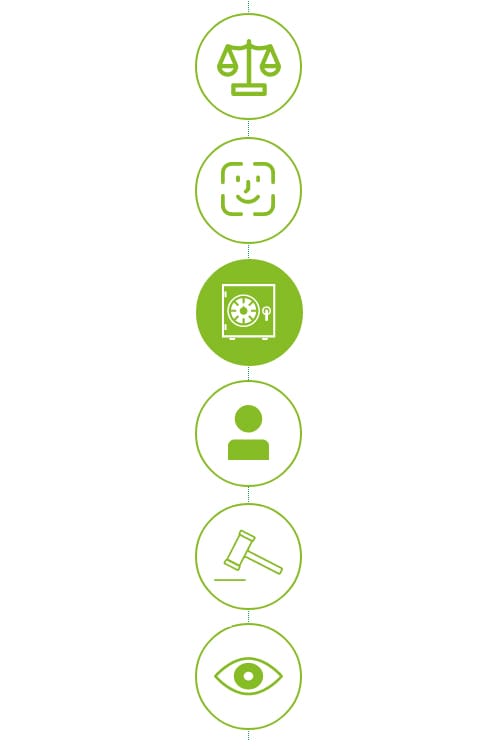
Accountable
With more traditional types of AI, a core ingredient for ethical decision making is the stakeholder’s capacity to understand the model, its function, and its outputs. Because an AI model cannot be meaningfully held accountable for its outputs, accountability is squarely a human domain. In some use cases, generative AI makes accountability a much thornier and more complicated matter.
In the near future, major companies may deploy an “AI spokesperson,” backed by the full suite of social and marketing tools, customer profiles, enterprise data, and more. They will be tuned to specific subjects (e.g., home improvement tips from a home goods retailer), they will be tweaked to express a personality, and eventually, they will become persistent, meaning they will recall interactions with individual users wherever they encounter them (e.g., social media, company website, support call centers).
How can the business direct the trustworthy behavior of a persistent AI personality that is operating at such an enormous scale that it eclipses the possibility for transparency and keeping a human in the loop? What happens if AI spokespeople from competing brands begin arguing on a social media site and one disparages the other? What happens if one AI spokesperson begins lying or deliberately encouraging the misuse of a competing product? What happens if one AI spokesperson mimics and pretends to be another?
Ultimately, the organization deploying the tool is accountable for its outputs and the consequences of those outputs. Whether the enterprise uses a model built in-house or purchases access through a vendor, there needs to be a clear link between the generative AI model and the business deploying it.

Responsible
Just because we can use generative AI for a given application does not always mean we should. Indeed, the sword of generative AI cuts both ways, and for all the enormous good it can be used to promote, generative AI use cases could also lead to significant harms and disruption. Imagine a scenario where a politician is running for office and an opponent group uses generative AI to simulate a hyper-realistic video of the candidate saying and doing untoward things. Without context, the voting populace may begin to doubt what is true. This injects confusion and political disruption, and more profoundly, it could undermine the government systems that are crucial to a healthy society.
Imagine a similar scenario on the global stage. Audio data could mimic a world leader threatening conflict. Translations could be augmented to misrepresent intentions. Videos could be created to show military conflict that is not actually occurring. And all of this can be done cheaply, in real time, personalized to the audience, and delivered at scale. In this confused space, the line between objective truth and generative AI-enabled deception blurs.
Yet, even when generative AI outputs are fruitful (or at least benign), there remain questions about responsible development and deployment. For example, consider that training, testing, and using generative AI models can lead to significant energy consumption, with implications for climate change and environmental sustainability. This consequence of generative AI deployment may not align with an organization’s goals for reducing their carbon footprint. In this way, the question of whether it is a responsible decision to develop and deploy a model depends on the organization and its priorities. What is judged to be a responsible deployment by one organization may not be judged the same by another. Enterprise leaders need to determine for themselves whether a generative AI use case is a responsible decision for their organization.
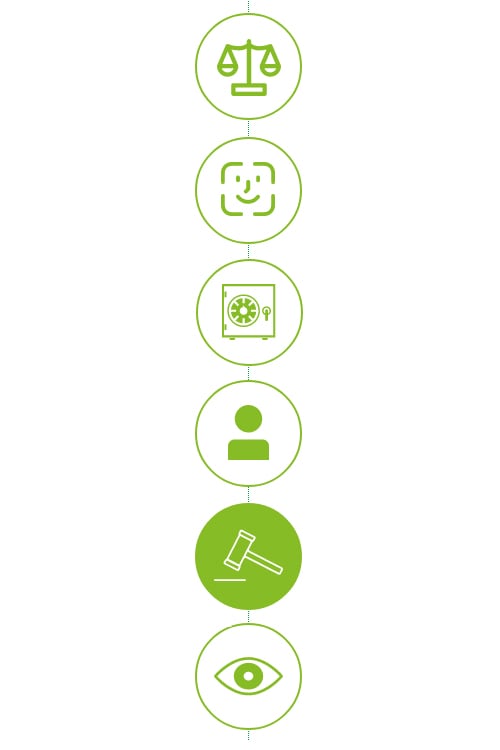
Privacy
The data used to train and test generative AI models may contain sensitive or personally identifiable information that needs to be obscured and protected. As with other types of AI, the organization needs to develop cohesive processes for managing the privacy of all stakeholders, including data providers, vendors, customers, and employees. As a part of this, the enterprise may turn to tactics such as removing personal data, using synthetic data, or even preventing end users from inputting personal data into the system.
There are also significant questions around generative AI-derived intellectual property. Copyright laws are generally concerned with guarding a creator’s economic and moral rights to their protected work. What happens when something is created by generative AI with minimal or no human involvement? Can that be copyrighted? For enterprises, consider how generative AI is used to create business-critical data (e.g., for product prototyping) and whether its derivations legally and solely belong to the organization.
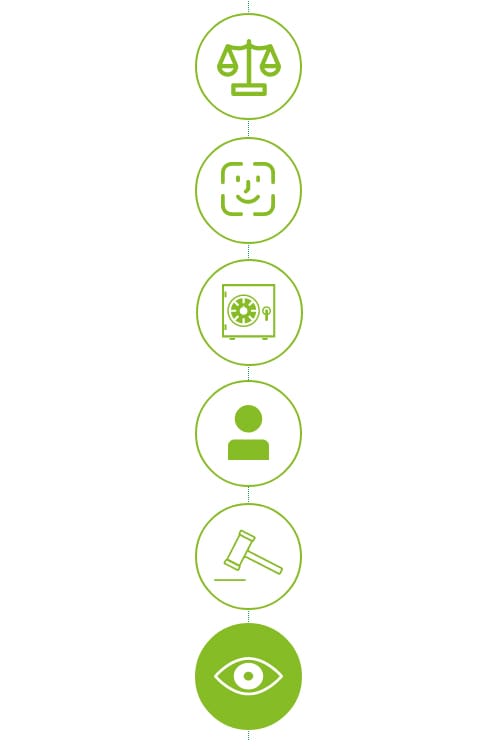
Considerations and tactics for trustworthy Generative AI
Effective, enterprise-wide model governance is not something that can be dismissed until negative consequences emerge, nor is it sufficient to take a “wait and see” approach as government rulemaking on generative AI evolves. Instead, given the potential consequences, businesses face a need to account for generative AI risks today and those yet to emerge as the technology matures.
Fortunately, just as the domains of AI trust hold true for generative AI models, so too does the prescription for governance. At its core, it is a matter of aligning people, processes, and technologies to promote risk mitigation and establish governance. With the workforce, the duty to identify and manage risk is shared throughout the organization among both technical and non-technical stakeholders.
To participate in generative AI governance, these stakeholders need a clear sense of roles and responsibilities, as well as workforce training opportunities to enhance their AI literacy and skills to better work with and alongside this technology. The enterprise may also create new roles and groups within the business, such as an AI ethics advisory board charged with overseeing and guiding the trustworthy use of generative AI. As a part of this, businesses can look to building diverse teams that help shape and govern AI with a multitude of perspectives and lived experiences.
Meanwhile, processes may need to be invented or augmented. Risk assessment and analysis should be baked into the entire generative AI lifecycle, with regular waypoints for stakeholder review and decision making. There are considerations for how user data inputs are stored, transferred, and leveraged to enhance or improve the model, which cuts across processes in practice areas in legal, compliance, and cybersecurity. And as regulatory bodies worldwide begin to establish rules for the use of generative AI, things like documented impartiality, model explainability, and data privacy will become even more important for AI programs.
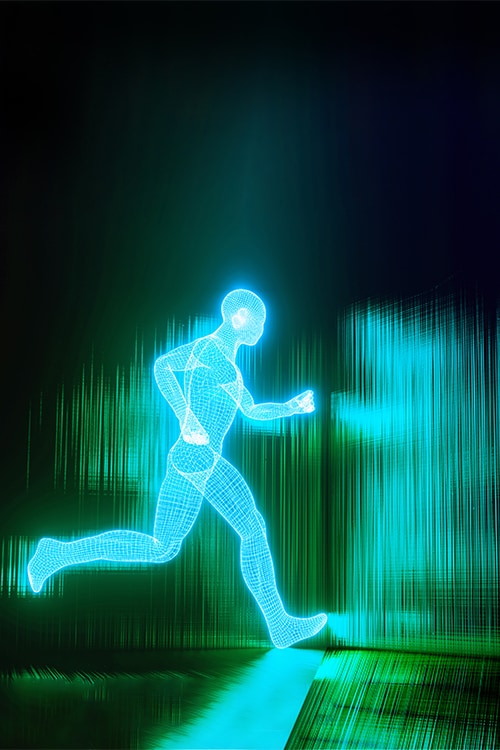
When it comes to technology, the “black box” problem of traditional AI is magnified greatly. Large language models, for example, can have billions of parameters, and understanding how and why a generative AI model determined its output may be far out of reach, even for technical stakeholders. Issues with transparency and explainability are compounded by the challenge of aligning generative AI outputs with enterprise priorities and values. To help promote model transparency and ongoing improvement, organizations may look to leverage technology platforms that help evaluate and track model performance, and assess, manage, and document each step of the AI lifecycle. This helps the enterprise evaluate whether an AI tool performs as intended and aligns with the relevant dimensions of trust.
To be sure, the risks associated with generative AI, and the work required to mitigate them, are significant. Yet, for many organizations, the risks of not embracing generative AI outweigh the risks the technology creates. Organizations across industries are exploring how to capitalize on generative AI capabilities, and as with many transformative technologies, standing still means falling behind.
The opportunity and challenge for businesses is to maximize the value they can extract from generative AI deployments while consistently governing the lifecycle and mitigating risks as they arise.
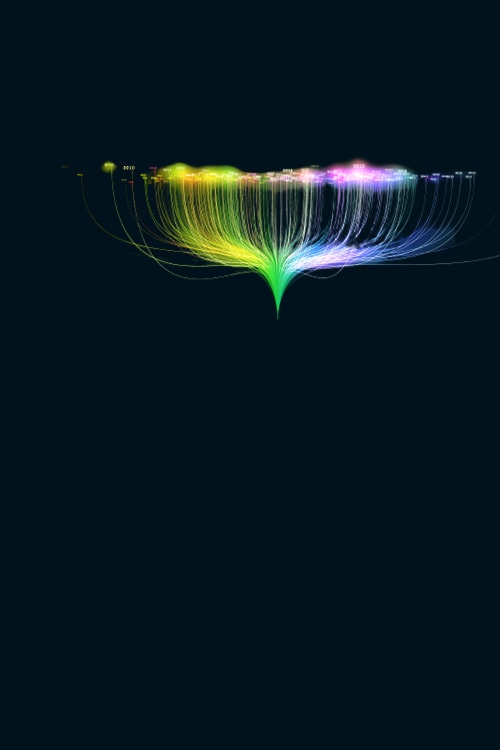