Deloitte AI Institute
Using AI in predictive maintenance to forecast the future
The value of machine learning in predictive maintenance
As the cost of capital may continue to rise and macroeconomic trends could signal challenges ahead, enterprise budgets may be pressured on all sides. Existing infrastructure could be asked to do more while supply chain disruption might persist. To address these challenges, organizations could try to extract the most bottom-line value from existing assets and investments. An opportunity may exist in better managing physical assets to help minimize downtime, maximize productivity, and save as much capital as possible in labor, materials, and expenditures.
Preventative maintenance, an approach to asset management, may no longer be enough in today’s globalized marketplace. Strategies such as scheduled maintenance checks and conditioned-based maintenance may not always be enough to confirm asset reliability in a fast-paced, complex environment for manufacturing, logistics, and operations. Whether the concern is cascading damage to the wider system, the quality of products, the safety of the process and facility, or other consequences from aging or failing assets, it may be important to build the capacity to help predict asset failure and help prevent it from occurring in the first place.
To better understand what is at stake and the options available, consider the many business-critical advantages that could flow out of predictive maintenance.
The multi-dimensional value in predictive maintenance
Industrial automation is growing rapidly with the development of IoT technologies, reduced cost of data storage/computing, and advancements in AI/ML capabilities. Yet, based on our experience, maintenance organizations have not been able to harness the power of these technologies beyond pilots.
Maintaining assets at its base may be a task of limiting and avoiding downtime and driving efficiency in maintenance could help maximize asset usage and keep operations moving. This applies to assets within facilities, like manufacturing plants, as well as assets in the field. Yet, the business value in effective maintenance may be bigger than asset uptime.
Business value of effective maintenance
Limiting fallout: By anticipating and avoiding a machine failure, the enterprise could prevent cascading impacts that could slow other operations and cause costly outages.
Elevating ROI: Preventing failure can extend the life of machines and assets, allowing the business to extract more value from existing investments.
Empowering the workforce: When failures are prevented, the maintenance workforce spends less time reacting to machine failure and more time anticipating and preventing future issues, which could make the best use of valuable human capital.
Supporting procurement: Knowing when a replacement part will be needed helps the procurement process, improving planning, timing, and sourcing in the face of ongoing supply chain interruptions. Additionally, this could help the business limit the amount of spare equipment kept on site as well as the amount of business capital tied up in parts that are not yet needed.
Improving safety: A failing machine can imperil employees as well as other assets and production overall. Predicting and preventing failures could improve the safety of enterprise operations.
Improving quality control: Assets that are heading toward failure may not operate to the desired specifications and can diminish product quality. Conversely, quality control may be helped through proactive intervention and maintenance.
Managing environmental impact: By more efficiently using materials used in maintenance, from replacement parts to shop supplies, waste is reduced, positively impacting the environmental footprint of operations.
Predictive maintenance could support these outcomes. The challenge may come in knowing which technologies and process changes can support predictive maintenance operations across the business.
Using AI to help forecast the future
Historically, maintenance schedules may have been either based around estimates of a machine’s lifetime and projected time to failure or based on recommendations from the original equipment manufacturer. To help improve maintenance operations, the enterprise can replace educated guesses with data-based knowledge about how an asset is performing and when it will degrade.
Getting to this level of predictive maintenance begins with incorporating additional data sources. Sensors can be added to key components to capture data points about how the asset is working. Other data sources that can help unlock value include procurement and enterprise resource planning (ERP) data, historical maintenance and repair data, production data, and ongoing reports from employees in the field.
When the data is consolidated and interpreted with AI-enabled signal processing, the result can be a deeper and more nuanced understanding of not just individual machines but the larger network of interdependent assets. By harnessing the collective knowledge of people, sensors, and systems, the business can use AI to analyze the information and output maintenance recommendations. These recommendations can be automatically prioritized, which may help optimize how the human workforce allocates its time. In a way, the AI solution could serve as an omnipresent maintenance employee helping the human workforce make better decisions about when and where to target operations.
As an example, Deloitte worked with a major logistics provider that was struggling with conveyance equipment in its distribution centers. By adding sensors onto the assets and pulling data from every facility into a cloud environment, the company was able to use analytics to identify the lifespan of equipment across the facility network and target maintenance interventions before a failure. The result was faster and more efficient operations, which translated into greater competitiveness in the marketplace
Bridging the gap to predictive maintenance
The value may be evident and the enabling technologies known, but shifting the enterprise from reactive maintenance to proactive and predictive operations can be a complex endeavor. A transformational project requires specialized skills and knowledge to architect the systems, design the sensor strategies, and create data pipelines from the edge into the cloud. It also might require machine learning engineers and data scientists to prepare and train the predictive models, as well as a workforce that is trained to make sense of the signals and push those predictive insights into a case management system or workflow.
Each of these tasks takes specialized skillsets and leading practices rooted in experience, some of which may not be available in house. Working with an organization, like Deloitte, can help expedite the process of orchestrating the data collection, inference engine and action engine, as well as provide the change management, documentation, and training needed to help the human workforce adopt and use predictive maintenance technologies.
To be sure, every business is different, just as every asset is different. A leading constellation of solutions and platforms could be somewhat different between enterprises, and a starting point is assessing maintenance maturity to identify where new data flows and AI analysis can begin improving operations. Transforming to predictive maintenance may not be an all-or-nothing proposition. Some organizations may test new capabilities in a pilot program. With evidence of how predictive maintenance can help improve quality, safety, workforce productivity, and asset uptime, the business could build momentum to seek other use cases, scale the program, and promote enterprise-wide enthusiasm and adoption.
In this era of humans working with intelligent machines, the race may be on to find value-driving AI applications that can help create competitive differentiators in the market. We may not need AI to predict the future of maintenance operations. The task may now be to make the targeted investments and decisions that can help make it real.
Get in touch
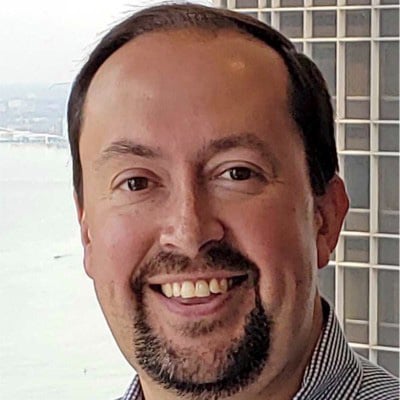
Derek D. Snaidauf
Principal | AI, Cyber, and Cloud
Deloitte Transactions and Business Analytics LLP
+1 (312) 898 0420
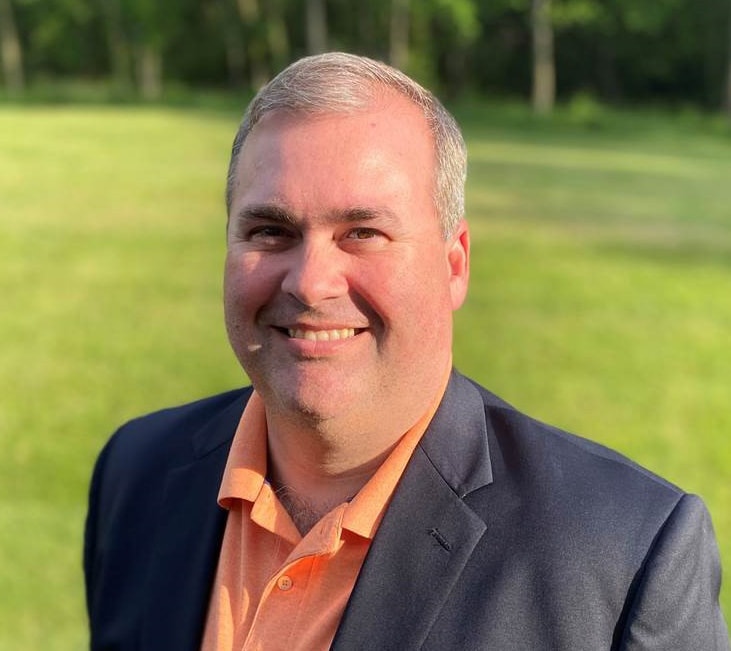
Edward Bowen
Managing Director, AI Center of Excellence
Deloitte Transactions and Business Analytics LLP
edbowen@deloitte.com
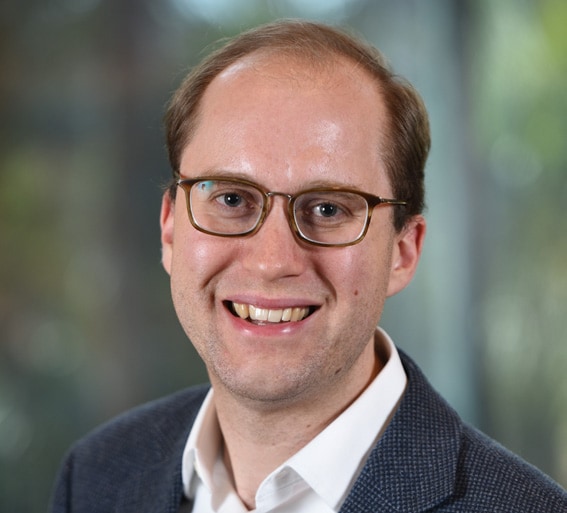
Don Williams
Senior Manager | Deloitte Risk and Financial Advisory
Analytics & Technology | Regulatory & Legal Support
Deloitte Transactions and Business Analytics LLP
+1 (202) 713-5040
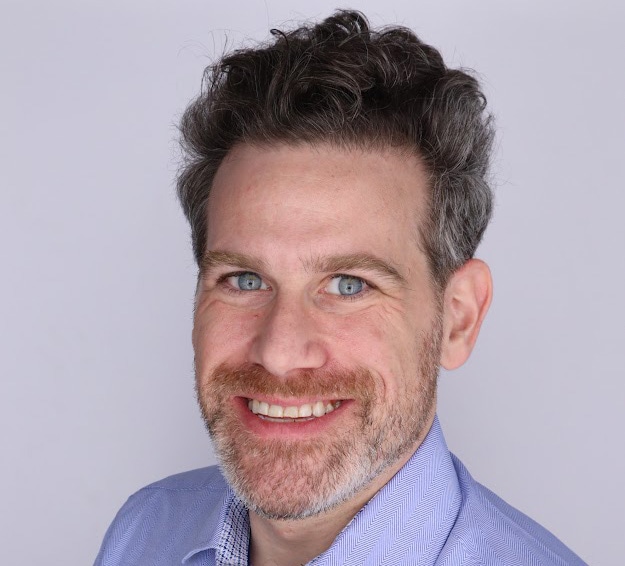
Ephraim Schoenbrun
Advisory Manager | Forensic Analytics
Deloitte Transactions & Business Analytics
+1 (212) 436 3889
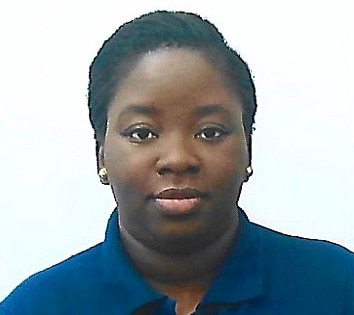
Hadi Diaby
Manager | Forensic Analytics
Analytics & Technology | Regulatory & Legal Support
Deloitte & Touche LLP
+1 (309) 433 3127
Let's talk