The evolution of forensic investigations has been saved
Perspectives
The evolution of forensic investigations
Integrating human and machine intelligence
Organizations can better identify and investigate attacks, as well as thwart future ones, by combining artificial intelligence (AI), machine learning, and statistical concepts of cognitive analytics with skilled forensic investigation of fraudster motives and methods. Such an approach can help investigators get to the bottom of the problem quicker and identify the root cause of incidents to improve their sensing capabilities and help prevent re-occurrence.
Explore content
- Current challenges of fraud investigation
- A path to integrated, analytics-driven fraud investigations
- View the infographic
- Get in touch
- Join the conversation
Current challenges of fraud investigation
Protecting data, intellectual property (IP), and finances has become an increasing priority at the board room level as fraudsters proliferate and constantly adapt to more sophisticated controls and monitoring. While most organizations are susceptible to seemingly boundless criminal ingenuity, those lacking antifraud controls are predictably worse off, suffering twice the median fraud losses of those with controls in place.
However, even organizations with antifraud controls can have their investigative efforts impeded by several factors.
- Reliance on rules-based testing is a primary culprit. Rules-based tests typically assess and monitor fraud risks across a single data set, giving only a yes or no answer.
- Information silos further impede analytics-aided investigative efforts. Organizations often struggle to balance the need for locally-tailored processes with the potential benefits of integrated data sharing, unintentionally creating barriers to investigative exploration as a result.
- The vast and growing volumes of unstructured data amassing in organizations, such as videos, images, emails, and text files.
- Often overmatched in the fraud wars. They rely on manual processes and ad-hoc data analysis, at significant dollar and time expense.
A path to integrated, analytics-driven fraud investigations
Cognitive data-driven approach starts with examining transaction data to identify abnormal gift purchases. This approach allows the data to tell investigators where to look for problems, unlike an intuition-driven approach that is purely based on their experience and knowledge.
An integrated, analytics-driven fraud investigation approach has several key dimensions:
- Analytics maturity. The ability to conduct an analytics-driven investigation begins with determining where an organization resides on a maturity model that captures the people, process, and tool dimensions of fraud analytics and forensics.
- Integrated data marts. The ability to integrate structured and unstructured data from internal and external sources into risk models is fundamental to an advanced analytics response.
- Risk-scoring of the entity rather than the transaction. Data-driven advanced analytics models incorporating text analytics and network analysis enable organizations to rank risks at the individual or entity level, rather than the transaction level.
- Application of predictive tools. Advanced analytics techniques, such as machine learning and cognitive computing.
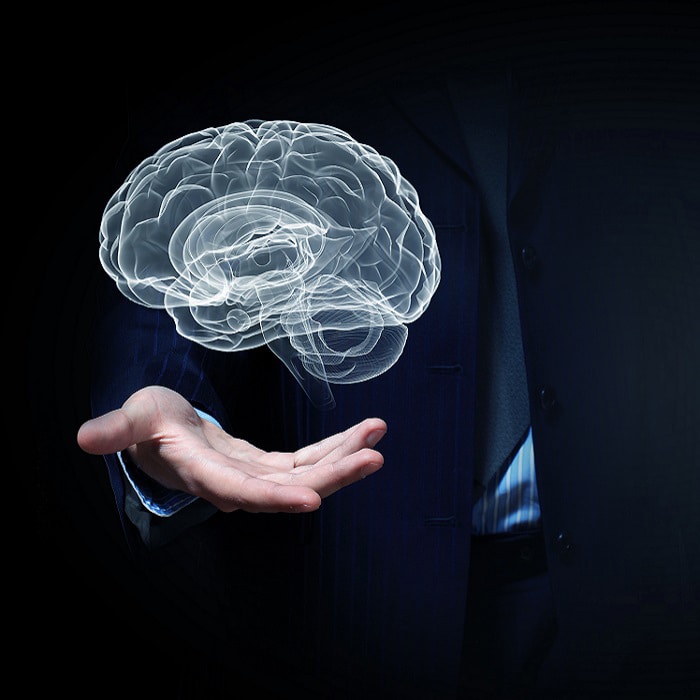
Recommendations
LIBOR reform
Repapering with a competitive edge
Analytics innovations in enterprise fraud management (EFM)
New digital defenses for new digital risks