HealthPrismTM
Health analytics for the public sector
This predictive analytics tool uses the largest drivers of health dataset, geospatial analytics, and AI to empower decision-makers to improve health outcomes for communities they serve.
HealthPrism data summary
Largest drivers of health dataset
covering 335 Million people in the US
Individual-level data with eight years
of history across 1,700 data variables
Data from over 50 large
commercially available datasets
(with the ability to integrate additional data)
Reveal deep insights about population health
Using advanced analytics techniques and one of the largest drivers of health datasets, HealthPrism provides health and human services agencies with the ability to surface disparities in large populations, enabling decision-makers across the health ecosystem to:
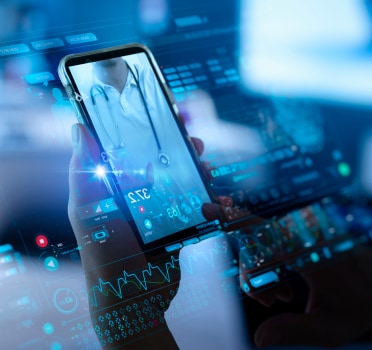
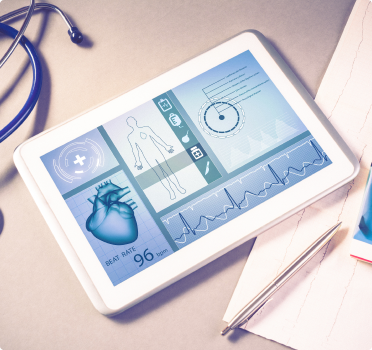
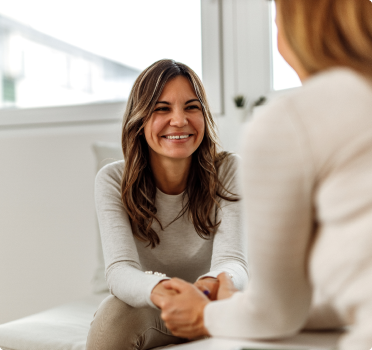
How HealthPrism works
Identify unique
subpopulations
Identify drivers of health that could influence health outcomes and pinpoint programs and interventions to lower risk.
Activate the community
Inform micro-tailored outreach campaigns by identifying optimal messaging strategies and modes of outreach.
Guide resource allocation
Find care deserts and populations facing barriers to healthy outcomes to deliver resources down to the neighborhood level.
Measure program effectiveness
Evaluate program and intervention efficacy using longitudinal data, gauging population changes over time.
HealthPrism in action: Explore our interactive dashboard
Despite ongoing community interventions and prevention efforts, fatal and non-fatal drug overdoses continue to rise. Using HealthPrism data, state and community leaders can explore the drivers of opioid use disorder and identify at-risk populations to tailor strategies that help curb this national issue.
This dashboard was created using HealthPrism data. See more information about HealthPrism data in the FAQ section.
Who can benefit from HealthPrism
State departments of health
State departments of Medicaid
Federal health agencies
Health nonprofit organizations
Commercial health care organizations
Frequently asked questions
- HealthPrism helps resolve data gaps commonly found in publicly available data sources (such as underreporting of unhoused populations) by compiling data from more than 50 data sources and using predictive modeling methods.
- HealthPrism gives the ability to look at multiple data variables at once, enabling users to segment broad populations by multiple variables to find unique subpopulations.
- Because HealthPrism has individual-level data, it can easily be integrated with your data at any level of aggregation.
HealthPrism uses semi-supervised machine learning (ML) methods to train explainable predictive models. It generates modeling datasets that represent the United States population with respect to legally protected groups. HealthPrism also extends bias checks to include representation across income groups and the Rural-Urban Continuum Codes. Additionally, each variable input into a model is examined for its distribution—with respect to age, income, the rural-urban continuum and other demographic factors—to help ensure that it does not act as a proxy for any of those variables. This bias awareness and examination can also influence the strategy for model selection in which only explainable and interpretable models are used to train and predict risk.
HealthPrism is committed to maintaining the privacy of personal data, protecting user data and maintaining transparency with strict controls around data usage:
- Secure and controlled data: HealthPrism is controlled, encrypted, and protected through a Confidential Information Management Plan (CIMP) and is FedRAMP ready.
- Nationwide opt-in/opt-out access: HealthPrism complies with the strictest data privacy act in the US, the California Consumer Privacy Protection (CCPA) Act. HealthPrism only includes data that individuals have elected to share and provides the option for individuals to opt out of our dataset.
- No data from scraping or monitoring: We use consensual data and never process data that has been sourced through internet data scraping or monitoring.
HealthPrism data reflects predictive estimates based on multiple demographic and consumer marketing data sources. Underlying data and risk estimates are updated on a regular basis (typically every one to two months, depending on the source). In other words, the data in this dashboard is updated on a consistently recurring basis as opposed to a real-time basis.
Populations that suffer disproportionately high rates of death and disease—such as racially and ethnically
diverse populations, people with disabilities, and rural populations—are underrepresented in some
health research,
and their status as part of those groups is often not recorded in critical
health care records. This lack of data is a serious barrier to advancing equity.
Race and ethnicity data are particularly essential to understanding and improving health equity.
However, this data on much of the US population is missing from many health-related datasets.
Race and ethnicity are
often poorly documented
in commercial health records like insurance claims. Additionally,
gaps in data and outdated information in public data sources like the US Census can hinder meaningful insights
into race and ethnicity. These gaps can prevent recognition very real health disparities and delay action to
improve equity.
To enable meaningful action on health equity, HealthPrism uses predictive models based on the Bayesian
Improved Surname and Geocoding Method
(BISG) to fill the gaps in missing race
and ethnicity data (this method is used by government agencies like the
Virginia Department of Health).
In addition, HealthPrism uses other data science methods to improve race and ethnicity predictive model accuracy.
HealthPrism includes self-reported race in which such data is available and displays predicted race where
no self-reported data is available.
Get in touch
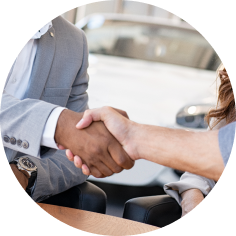
Let's start a conversation about what HealthPrism can do for your organization:
healthprismcontact@deloitte.comContact Us
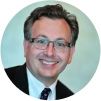
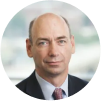
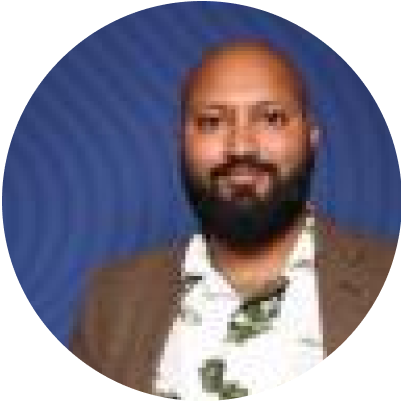