Managing algorithmic risks has been saved
Perspectives
Managing algorithmic risks
Safeguarding the use of complex algorithms and machine learning
Increasingly, complex algorithms and machine learning-based systems are being used to achieve business goals, accelerate performance, and create differentiation. But they often operate like black boxes for decision making, and are not controlled appropriately, though they are vulnerable to a variety of risks. Learn to harness the power of complex algorithms while managing the accompanying risks with a robust algorithmic risk management framework.
Explore content
- The algorithmic revolution is here
- When algorithms go wrong
- What are algorithmic risks?
- Why are algorithmic risks gaining prominence today?
- How to manage algorithmic risks
- Is your organization ready to manage algorithmic risks?
- What's next
- Get in touch
- Join the conversation
The algorithmic revolution is here
The rise of advanced data analytics and cognitive technologies has led to an explosion in the use of algorithms across a range of purposes, industries, and business functions. Decisions that have a profound impact on individuals are being influenced by these algorithms—including what information individuals are exposed to, what jobs they’re offered, whether their loan applications are approved, what medical treatment their doctors recommend, and even their treatment in the judicial system.
What’s more, dramatically increasing complexity is fundamentally turning algorithms into inscrutable black boxes of decision making. An aura of objectivity and infallibility may be ascribed to algorithms. But these black boxes are vulnerable to risks, such as accidental or intentional biases, errors, and frauds—raising the question of how to “trust” algorithmic systems.
Embracing this complexity and establishing mechanisms to manage the associated risks will go a long way toward effectively harnessing the power of algorithms. Organizations that adapt a risk-aware mind-set will have an opportunity to use algorithms to lead in the marketplace, better navigate the regulatory environment, and disrupt their industries through innovation.
When algorithms go wrong
Business spending on cognitive technologies has been growing rapidly. And it’s expected to continue at a five-year compound annual growth rate of 55 percent to nearly $47 billion by 2020, paving the way for even broader use of machine learning-based algorithms. Going forward, these algorithms will be powering many of the IoT-based smart applications across sectors.
But instances of algorithms going wrong or being misused have also increased significantly. Here are just a few:
- During the 2016 Brexit referendum, algorithms were blamed for the flash crash of the British pound by six percent in a matter of two minutes.
- Investigations have found that the algorithm used by criminal justice systems across the United States to predict recidivism rates is biased against certain racial classes.
- Researchers have found erroneous statistical assumptions and bugs in functional magnetic-resonance imaging (fMRI) technology, which raised questions about the validity of many brain studies.
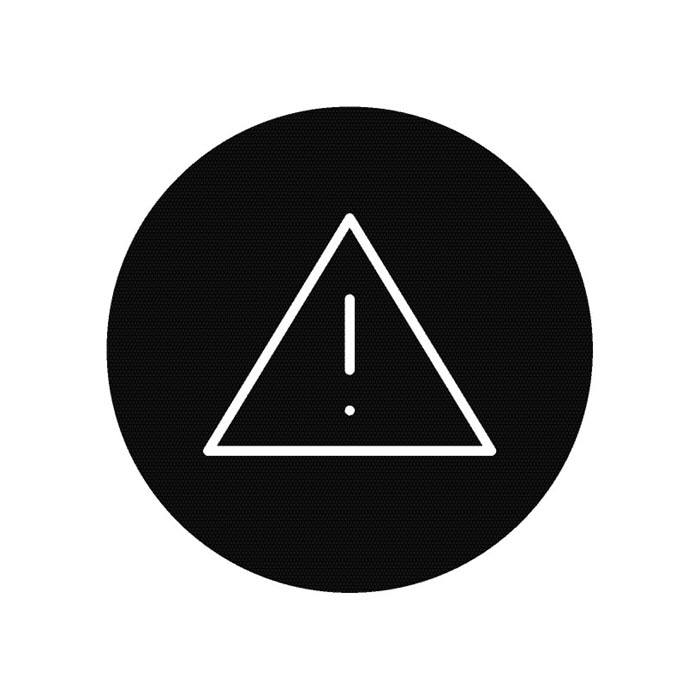
What are algorithmic risks?
Algorithmic risks arise from the use of data analytics and cognitive technology-based software algorithms in various automated and semi-automated decision-making environments. Three areas in the algorithm life cycle have unique risk vulnerabilities:
- Input data is vulnerable to risks, such as biases in the data used for training; incomplete, outdated, or irrelevant data; insufficiently large and diverse sample size; inappropriate data-collection techniques; and a mismatch between the data used for training the algorithm and the actual input data during operations.
- Algorithm design is vulnerable to risks, such as biased logic, flawed assumptions or judgments, inappropriate modeling techniques, coding errors, and identifying spurious patterns in the training data.
- Output decisions are vulnerable to risks, such as incorrect interpretation of the output, inappropriate use of the output, and disregard of the underlying assumptions.
The immediate fallouts of algorithmic risks can include inappropriate and potential illegal decisions. And they can affect a range of functions, such as finance, sales and marketing, operations, risk management, information technology, and human resources.
Algorithms operate at faster speeds in fully automated environments, and they become increasingly volatile as algorithms interact with other algorithms or social media platforms. Therefore, algorithmic risks can quickly get out of hand.
Algorithmic risks can also carry broader and long-term implications across a range of risks, including reputation, financial, operational, regulatory, technology, and strategic risks. Given the potential for such long-term negative implications, it’s imperative that algorithmic risks be appropriately and effectively managed.

Why are algorithmic risks gaining prominence today?
The growing prominence of algorithmic risks can be attributed to the following factors:
- Algorithms are becoming pervasive
- Machine learning techniques are evolving
- Algorithms are becoming more powerful
- Algorithms are becoming more opaque
- Algorithms are becoming targets of hacking

How to manage algorithmic risks
Conventional risk management approaches aren’t designed for managing risks associated with machine learning or algorithm-based decision-making systems. This is due to the complexity, unpredictability, and proprietary nature of algorithms, as well as the lack of standards in this space.
Three factors differentiate algorithmic risk management from traditional risk management:
- Algorithms are typically based on proprietary data, models, and techniques
- Algorithms are complex, unpredictable, and difficult to explain
- There’s a lack of standards and regulations that apply to algorithms
To effectively manage algorithmic risks, there’s a need to modernize traditional risk-management frameworks. Organizations should develop and adopt new approaches that are built on strong foundations of enterprise risk management and aligned with leading practices and regulatory requirements. One approach, along with its specific elements, is shown below.
Is your organization ready to manage algorithmic risks?
A good starting point for implementing an algorithmic risk management framework is to ask important questions about the preparedness of your organization to manage algorithmic risks. For example:
- Does your organization have a good handle on where algorithms are deployed?
- Have you evaluated the potential impact should those algorithms function improperly?
- Does senior management within your organization understand the need to manage algorithmic risks?
- Do you have a clearly established governance structure for overseeing the risks emanating from algorithms?
- Do you have a program in place to manage these risks? If so, are you continuously enhancing the program over time as technologies and requirements evolve?

What’s next
Managing algorithmic complexity can be an opportunity to lead, navigate, and disrupt in your industry. Contact us to discuss how the ideas presented in this paper apply to your organization. And how you can begin to open the algorithmic black box and manage the risks hidden within.
To read the full report, download Managing algorithmic risks: Safeguarding the use of complex algorithms and machine learning.
Recommendations
Future of risk in the digital era
Transformative change. Disruptive risk.
Evolving governance and controls for automation
Future of risk in the digital era