Overcoming data challenges in forensic investigations has been saved
Perspectives
Overcoming data challenges in forensic investigations
The foundation for integrated human and machine intelligence
Organizations across industries, and regulators themselves, are starting to use integrated, data-driven analytics approaches to identify potentially fraudulent transactions. Those that do not could potentially rapidly fall behind and face increasing financial, reputational, legal, and regulatory risks.
Explore content
- Data challenges abound
- Data considerations for analytics-driven fraud risk management
- View the infographic
- Get in touch
- Join the conversation
Data challenges abound
An array of factors can contribute to gaps and shortcomings in monitoring fraud and
Vast amounts of data. Companies now electronically collect, process, and store more information than was imaginable even 10 years ago. And while the growth in data volume is impressive, even more so is the expanding variety of data sources generating that volume, including personal and business mobile devices, Internet of Things-connected devices, and social media platforms.
Inadequate capture and storage. Legacy systems were often designed to capture information for a specific purpose, so the data available may not be rich enough for meaningful analytics. For example, transaction time stamps and the identities of employees performing transactions might not be captured.
Limited data accessibility. A company with decentralized operations and data sources that are siloed by geographies and departments may lack a master system to consolidate data globally, inhibiting cross-correlation.
Inadequate skillsets to process and analyze big data. When data volumes are small, basic analytical skills and spreadsheet programs might be adequate to handle preliminary analysis of structured data from enterprise systems and other software applications, as well as unstructured data such as emails, texts, and voice recordings. But when that volume grows into the millions, analysis can require technology, advanced analytics, and forensic skills that aren't readily available within many organizations.
Static reporting designed for business as usual. Legal, compliance, and internal audit teams may encounter barriers to gathering data from sources such as the finance, information technology (IT), procurement, and sales departments. Standard reports from those groups may provide only limited information; for example, in the context of procurement, identifying information such as a vendor contact name, address, and phone number might not exist in a standard vendor report, which could limit the ability to compare vendor contact information to employee data to determine potential overlap.
Lack of diverse data to correlate findings. Companies may not adequately explore external data sources, such as third-party reporting databases and social media, to capture a comprehensive view of the fraud risk presented by a company's supply chain, sales channel, and employees.
Data considerations for analytics-driven fraud risk management
Organizations can take several steps to prepare an effective foundation for analytics-driven investigations and fraud monitoring:
Involve stakeholders in building the transformation roadmap. Specific areas of a company may be primed and ready to undertake analytics-driven fraud risk management, but others need in on the plans, too. Internal audit, legal, compliance, IT, and the businesses can all have roles and interest in the analytics efforts. Discussions with relevant stakeholders can identify synergies and ways to leverage technologies in use elsewhere in the organization.
Centralize as much data as possible to support fraud monitoring. While centralizing all enterprise data would be the Holy Grail for the fight against fraud, it may not be realistic in many organizations today due to disparate data sources, geographic locations, and gaps in systems integration. Still, emphasis should be placed on bringing as much data together as possible to maintain data integrity, consistency, and control and for enhanced fraud monitoring, analysis, and insights.
Establish secure, structured access to data. A compliance department planning to conduct analytics can benefit by defining early on how data will be handled, where it will be stored, and who will be allowed access to it. Considerations include needed safeguards against breaches and policies and procedures for treatment of personally identifiable information and other sensitive data.
Incorporate relevant external data. External data can be brought into the centralized repository to cross-correlate with internal data.
Begin to lay a solid technology foundation. It is important to plan for investment in technology and software applications, as part of an overall enterprise solution, that can support effective data collection and analysis for fraud monitoring and to leverage the same data for multiple purposes. The technology should be scalable so both structured and unstructured enterprise data can be included in the analysis.
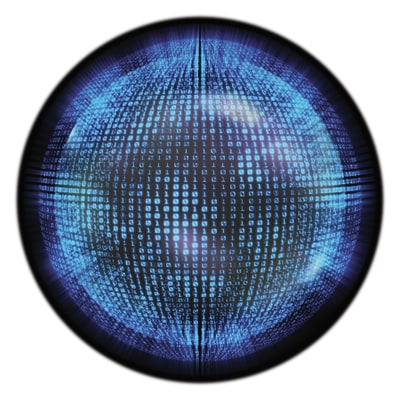
Recommendations
Cognitive computing applications for risk management
Better decision making with artificial intelligence
Tech-Enabled Investigations Spark Experience
Harnessing investigation capabilities for accelerated performance