The evolution of forensic investigations series has been saved
Perspectives
The evolution of forensic investigations series
An analytics-driven approach to fighting fraud
Forensic analytics is a critical capability in the future of investigations. Our five-part series explores analytics-driven fraud fighting approach, the need for available and accurate data, technologies required to extract data and realize its value, and continuous monitoring of transactions and activities, a process that produces invaluable results.
Explore content
- The evolution of forensic investigations
- Overcoming data challenges in forensic investigations
- Technology challenges in analytics-driven investigations
- Continuous fraud monitoring
- Forensic analytics in fraud investigations
Integrating human and machine intelligence
Organizations can better identify and investigate attacks, as well as thwart future ones, by combining artificial intelligence (AI), machine learning, and statistical concepts of cognitive analytics with skilled forensic investigation of fraudster motives and methods. Such an approach can help investigators get to the bottom of the problem quicker and identify the root cause of incidents to improve their sensing capabilities and help prevent re-occurrence.
An integrated, analytics-driven fraud investigation approach has several key dimensions:
- Analytics maturity. The ability to conduct an analytics-driven investigation
- Integrated data marts. The ability to integrate structured and unstructured data from internal and external sources into risk models is fundamental to an advanced analytics response.
- Risk-scoring of the entity rather than the transaction. Data-driven advanced analytics models incorporating text analytics and network analysis enable organizations to rank risks at the individual or entity level, rather than the transaction level.
- Application of predictive tools. Advanced analytics techniques, such as machine learning and cognitive computing.
Overcoming data challenges in forensic investigations
Organizations across industries, and regulators
Organizations can take several steps to prepare an effective foundation for analytics-driven investigations and fraud monitoring:
- Involve stakeholders in building the transformation roadmap. Discussions with relevant stakeholders can identify synergies and ways to leverage technologies in use elsewhere in the organization.
- Centralize as much data as possible to support fraud monitoring. Emphasis should be placed on bringing as much data together as possible to maintain data integrity, consistency, and control and for enhanced fraud monitoring, analysis, and insights.
- Establish secure, structured access to data. Considerations include needed safeguards against breaches and policies and procedures for treatment of personally identifiable information and other sensitive data.
- Incorporate relevant external data. External data can be brought into the centralized repository to cross-correlate with internal data.
- Begin to lay a solid technology foundation. Technology should be scalable so both structured and unstructured enterprise data can be included in the analysis.
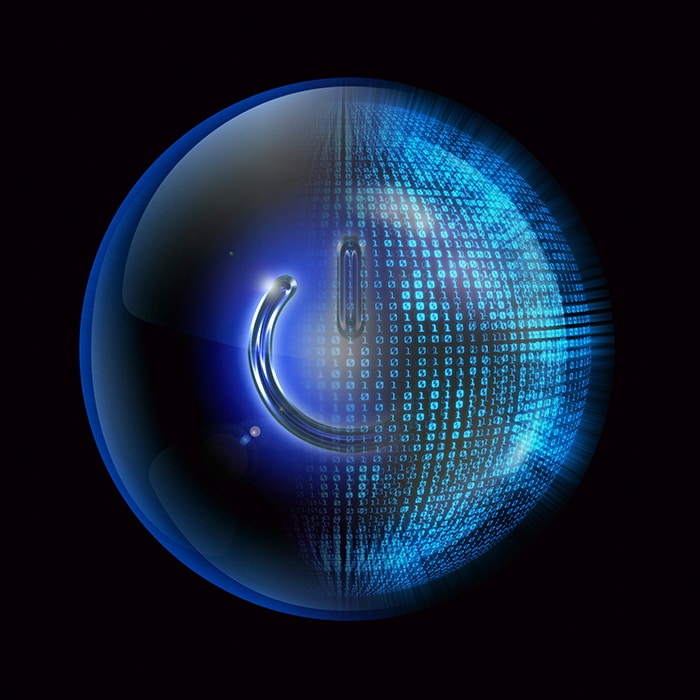
Technology challenges in analytics-driven investigations
Fraud can be as simple as intentionally making a duplicate payment. Or, it can be highly sophisticated, as fraudsters execute an ingenious play of intertwined transactions and third-party chicanery. However slick the scheme, fraud has been a persistent drain on an organization’s assets and a threat to people’s livelihoods. As perpetrators expand their larcenous repertoire, organizations across industries are starting to use integrated, data-driven analytics approaches to identify potentially fraudulent transactions.
As legal and compliance teams address the challenges described nearby, they can benefit from understanding some of the basic components of an integrated, data-driven analytics solution:
- Data management. Core functionality includes the architecture, protection, and policies and procedures associated with maintaining an organization’s data.
- Data and text mining. Core functionality can include anomaly or outlier detection, and predictive analytics to identify similarities based on known instances of fraud.
- Case management. Core functionality can include executive dashboards, calculated metrics, investigative lens, including focal entity and trending; flexible adjustment of requirements; system-based workflow; and a well-documented and communicated escalation process.
- Robotic process automation (RPA). Areas of potentially effective implementation of RPA include document review, customer research, and elements of third-party due diligence.
Continuous fraud monitoring
Organizations that use technology to monitor for potential risks, as well as analytics to identify new emerging threats, may be better positioned to mitigate the blind spots in their fraud defenses and address the risks of being blindsided financially, operationally, and legally.
Rather than relying on rules, analytics produce new insights driven by what the data is showing. Attention to several considerations can help an organization generate greater value from its monitoring activities:
- Embrace the deterrent effect. The mere existence of monitoring, properly communicated, can help nurture compliance with protocols, policies, and guidelines.
- Keep it in house. Data can be analyzed more easily on a continuous basis, and the in-house personnel can learn both how the solution works and how to maintain it.
- Customize monitoring to specific risks. Understanding trends and tailoring fraud solutions to specific organizational characteristics and situations, with business unit involvement, can help capture greater value from monitoring activities.
- Capitalize on available resources. Some of the tools needed to conduct monitoring may already exist within the organization in areas such as finance and supply chain. Opportunities may exist to leverage these investments for risk management.
- Use a range of approaches. Different risks can require different analytical tools. Unsupervised modeling creates statistical profiles of normal transactions or entities and identifies outliers from these profiles.
- Involve stakeholders. Risk management is no longer just the responsibility of internal audit and compliance. Business units and other functions have roles to play in identifying, understanding, and addressing fraud risks.
- Focus the effort. Rather than casting a wide net, consider conducting a focused, specific proof of concept to understand how a solution works and the value it could potentially provide.
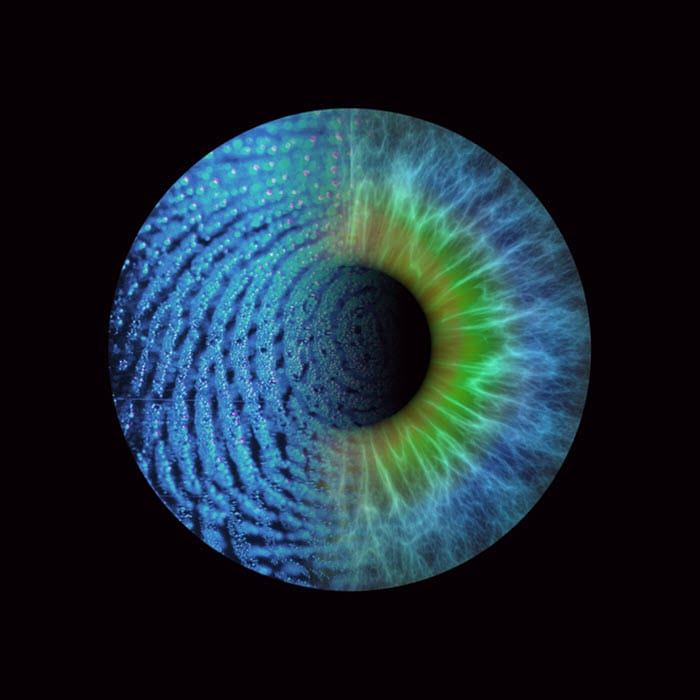
Forensic analytics in fraud investigations
Forensic analytics combines advanced analytics with forensic accounting and investigative techniques to identify potential rare events of consequence—needles in the massive haystacks of data and information that can signal trouble in the making. Urgently needed to meet growing regulatory and customer demands for fraud mitigation, forensic analytics can reveal signals of emerging risks months or even years earlier than possible otherwise.
Several methods warrant consideration in developing and applying forensic analytics:
- Training and self-learning. Analytics can learn from a variety of data sources, such as risk issues the organization has confronted in the past.
- Backtesting. Organizations can scientifically test forensic analytics performance in determining whether to use it.
- Iterative approach. As a forensic analytics solution is being implemented, models can be iteratively developed, adapted, and scaled so they respond to new and evolving fraud patterns, and at the same time, continually gain a broader view of the risks an enterprise may face.
- Feedback and continuous improvement. Once the forensic analytics solution is in place, its effectiveness can be continually improved by incorporating feedback from results of each investigation, from the continually growing body of forensic accounting and investigation knowledge and insight, and from the input of stakeholders across the enterprise.
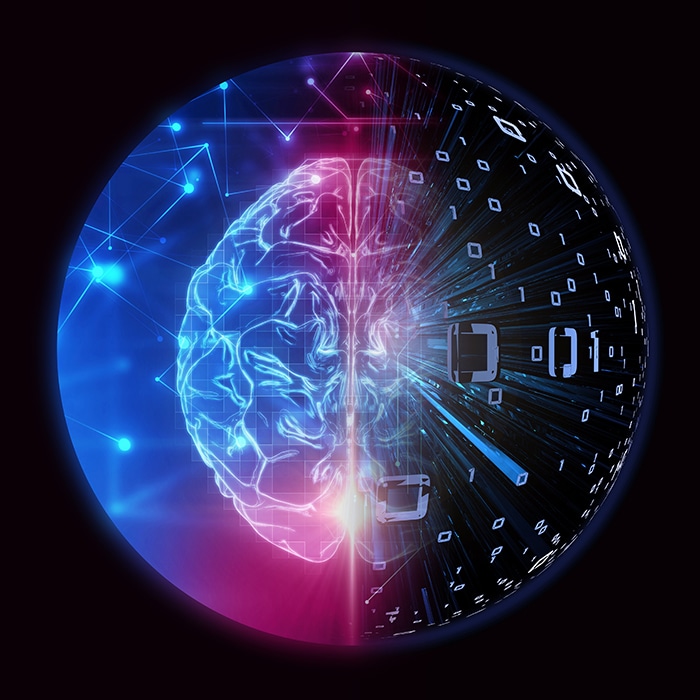
Archive
Protecting data, intellectual property, and finances
Learn more by downloading The evolution of forensic investigations: Integrating human and machine intelligence.
Recommendations
Cognitive computing applications for risk management
Better decision making with artificial intelligence
Tech-Enabled Investigations Spark Experience
Harnessing investigation capabilities for accelerated performance