Technology challenges in analytics-driven investigations has been saved
Perspectives
Technology challenges in analytics-driven investigations
Building the engine of integrated human and machine intelligence
Fraud can be as simple as intentionally making a duplicate payment. Or, it can be highly sophisticated, as fraudsters execute an ingenious play of intertwined transactions and third-party chicanery. However slick the scheme, fraud has been a persistent drain on an organization’s assets and a threat to people’s livelihoods. As perpetrators expand their larcenous repertoire, organizations across industries are starting to use integrated, data-driven analytics approaches to identify potentially fraudulent transactions.
Explore content
- Technology challenges in fraud investigations
- Solution component snapshot
- View the infographic
- Get in touch
- Join the conversation
Technology challenges in fraud investigations
Advanced analytics are making inroads into fraud investigations, but these are still early days. Legal and compliance organizations continue to use various legacy systems to perform data-intensive reviews. Analytics use cases tend to be ad-hoc ventures, typically performed by vendors. Tools are still maturing, a state that complicates long-term planning and investments.
A legal or compliance team that aims to elevate its fraud-fighting analytics technology capabilities can expect to encounter several challenges in the effort:
- Existing technology may not be adequate, and replacing it isn't easy.
- Current operating structures don't (yet) align with the tools.
- Investigation professionals may not know how to use, or may resist using, new technology.
- Outsourcing can lock the organization into a vendor solution.
- Data needs to be analyzed and interpreted.
Solution component snapshot
As legal and compliance teams address the challenges described nearby, they can benefit from understanding some of the basic components of an integrated, data-driven analytics solution:
- Data management. Core functionality includes the architecture, protection, and policies and procedures associated with maintaining an organization’s data.
- Data and text mining. Core functionality can include anomaly or outlier detection, and predictive analytics to identify similarities based on known instances of fraud.
- Case management. Core functionality can include executive dashboards, calculated metrics, investigative lens, including focal entity and trending; flexible adjustment of requirements; system-based workflow; and a well-documented and communicated escalation process.
- Robotic process automation (RPA). Areas of potentially effective implementation of RPA include document review, customer research, and elements of third-party due diligence.
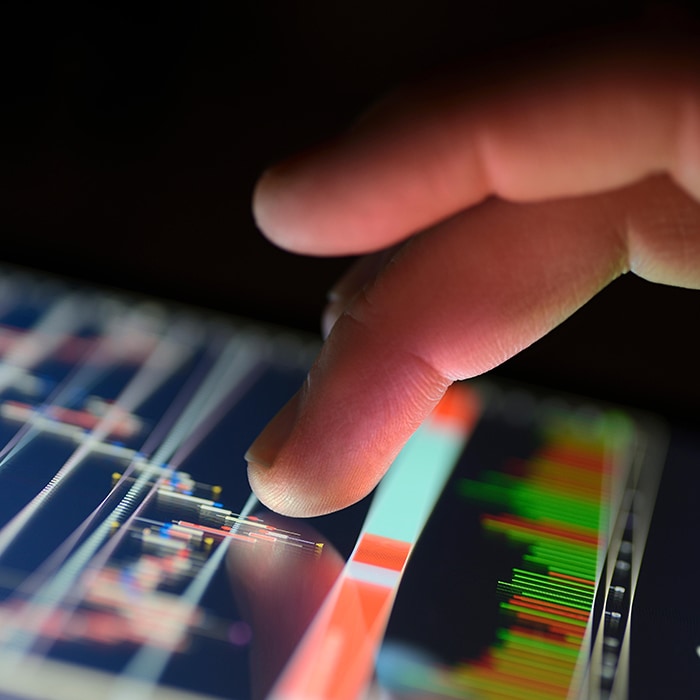
Recommendations
Cognitive computing applications for risk management
Better decision making with artificial intelligence
Five insights into anti-corruption compliance programs
Revisiting the FCPA